- [ ] Evaluating the Ideal Chunk Size for a RAG System using LlamaIndex
- https://blog.llamaindex.ai/evaluating-the-ideal-chunk-size-for-a-rag-system-using-llamaindex-6207e5d3fec5
- Chunking Strategies for LLM Applications https://www.pinecone.io/learn/chunking-strategies/
* 5 Levels Of Text Splitting/Chunking https://twitter.com/GregKamradt/status/1699465826485862543
* 案例 Langchain HTMLHeaderTextSplitter 加上 [[Self-Querying Retriever]]
* https://blog.langchain.dev/a-chunk-by-any-other-name/
* How to Chunk Text Data — A Comparative Analysis (2023/6/20)
* https://towardsdatascience.com/how-to-chunk-text-data-a-comparative-analysis-3858c4a0997a
* How Chunk Sizes Affect Semantic Retrieval Results https://ai.plainenglish.io/investigating-chunk-size-on-semantic-results-b465867d8ca1 2024/3/11
* Chunking https://www.rungalileo.io/blog/mastering-rag-advanced-chunking-techniques-for-llm-applications
* https://twitter.com/llama_index/status/1773522853939577243 (2024/3/29)
* 某種 a neat trick of both semantic chunking AND clustering
* Mastering RAG: Advanced Chunking Techniques for LLM Applications (2204/2/23)
* https://www.galileo.ai/blog/mastering-rag-advanced-chunking-techniques-for-llm-applications
* Advanced RAG 05: Exploring Semantic Chunking (2024/2/28)
* https://pub.towardsai.net/advanced-rag-05-exploring-semantic-chunking-97c12af20a4d
* Advanced RAG series: Indexing (2024/3/1)
* https://div.beehiiv.com/p/advanced-rag-series-indexing
* RAG in Production: Chunking Decisions (2024/4/7)
* https://pub.towardsai.net/rag-in-production-chunking-decisions-96a214dbbdc6
* How to Optimize Chunk Size for RAG in Production? (2024/5/13)
* https://pub.towardsai.net/how-to-optimize-chunk-sizes-for-rag-in-production-fae9019796b6
* https://x.com/llama_index/status/1792354714648211648
* Evaluating Chunking Strategies for Retrieval
* https://research.trychroma.com/evaluating-chunking (2024/7/3)
* 新提出 ClusterSemanticChunker 和 LLMSemanticChunker 效果好
* 我喜歡 LLMSemanticChunker 法
* prompt: https://github.com/brandonstarxel/chunking_evaluation/blob/main/chunking_evaluation/chunking/llm_semantic_chunker.py
* Chunking for RAG: best practices (2024/7/17)
* https://unstructured.io/blog/chunking-for-rag-best-practices
* The Art of Chunking: Boosting AI Performance in RAG Architectures (2024/8/18)
* https://towardsdatascience.com/the-art-of-chunking-boosting-ai-performance-in-rag-architectures-acdbdb8bdc2b
* 快速摘要 https://x.com/helloiamleonie/status/1838760385224089769 (2024/9/25)
* 5 Chunking Strategies For RAG
* https://blog.dailydoseofds.com/p/5-chunking-strategies-for-rag
* paper: Is Semantic Chunking Worth the Computational Cost?
* https://arxiv.org/abs/2410.13070
* 語義分塊相關的計算成本並未因一致的性能提升而得到合理化
* https://x.com/LargitData1/status/1857065872470188065 (2024/11/14)
## Sub-Document Summaries
https://twitter.com/llama_index/status/1761793821422264757 2024/2/26
將整份文件 或是 章節的摘要,也塞到 chunk 裡面,以改進上下文效能
https://twitter.com/jerryjliu0/status/1763728851568566474 2024/3/2
在 [[dsRAG 和 spRAG]] 中也有提到這招
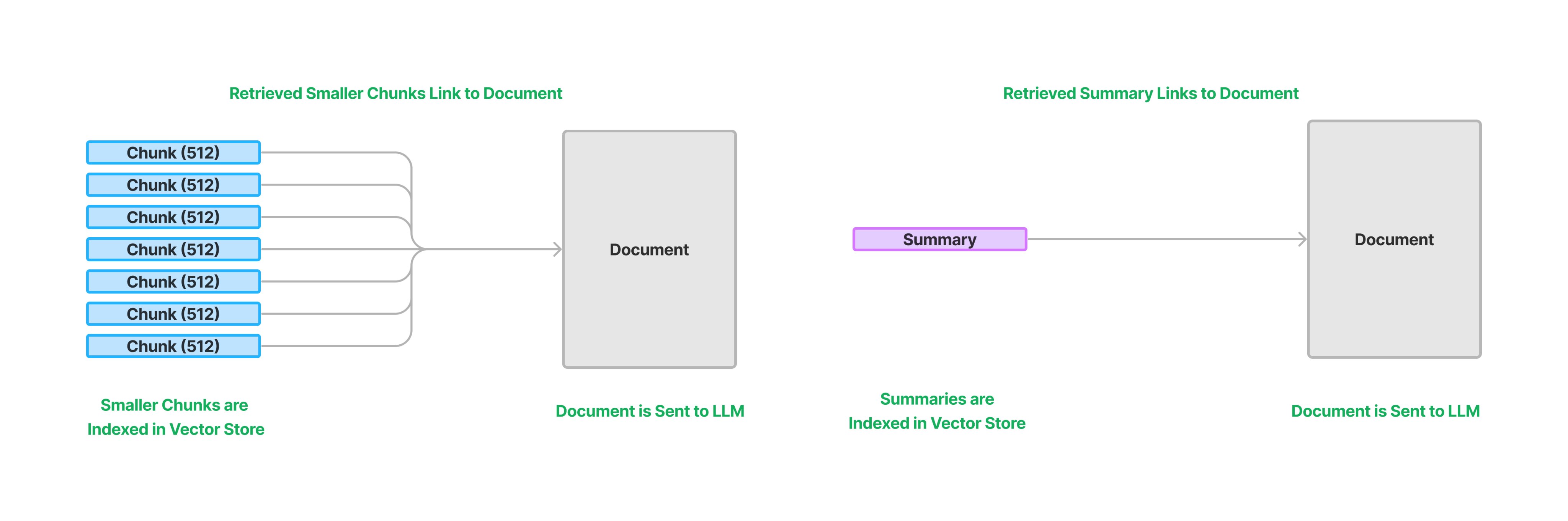
## The 5 Levels Of Text Splitting For Retrieval 影片
* https://www.youtube.com/watch?v=8OJC21T2SL4
* https://github.com/FullStackRetrieval-com/RetrievalTutorials/blob/main/5_Levels_Of_Text_Splitting.ipynb
* https://twitter.com/GregKamradt/status/1745467853799874969
* https://twitter.com/jerryjliu0/status/1745486856291266821
* https://twitter.com/dotey/status/1745585434107723983
* Level 1: Character Split
* Level 2: Recursive Character Split
* 以上用 langchain 跟 llamaindex 示範
* Level 3: Document Specific Splitting
* 針對 markdown, html, python code, javascript 切
* 針對 PDFs with Tables
* 用了 unstructured library 的 hi_res mode
* 使用 infer_table_structure: table 被轉成 HTML string
* 使用 extract_images_in_pdf: image 用了 GPT-4V 做文字摘要,然後再轉成 embeddings
* chunking 策略取決你的文件格式
* Level 4: Semantic Splitting (With Embeddings)
* 每句話都做 embedding,然後比較相似度
* 方法一: 做 clustering 加上位置獎勵
* 方法二: 用來找句子間的 breakpoint (接著demo這招)
* 並且分組用 window sentence: 1,2,3 和 2,3,4 和 3,4,5 .... 分組
* langchain 實作: https://python.langchain.com/docs/modules/data_connection/document_transformers/semantic-chunker
* Level 5: Agentic Splitting
* 先用 [[Proposition-Based Retrieval]] 處理轉成 propositions
* https://smith.langchain.com/hub/wfh/proposal-indexing?organizationId=50995362-9ea0-4378-ad97-b4edae2f9f22
* 迭代 propositions 累加,用 LLM 判斷是否屬於同一個 chunk,然後組成 chunk
* Multi-Vector Indexing
* Summaries
* Hypothetical questions
* Child Documents
* Graph Structure
### ChunkViz
https://chunkviz.up.railway.app/
## langchain
* https://zilliz.com/blog/experimenting-with-different-chunking-strategies-via-langchain (2023/10/24)
* 做了不同 chunk 大小的實驗,但似乎沒有評估啊???!!!
* 有拆出獨立的套件 https://pypi.org/project/langchain-text-splitters/
* https://python.langchain.com/docs/concepts/text_splitters/
## llamaindex
"try everything" approach
https://twitter.com/jerryjliu0/status/1745249025425863053
## Unstructured
chunking_strategy=by_title
https://medium.com/unstructured-io/rag-isnt-so-easy-why-llm-apps-are-challenging-and-how-unstructured-can-help-8daaf859c615
## Chonkie
* https://generativeai.pub/text-chunking-for-rag-systems-with-chonkie-d609d0eef55c
* https://github.com/chonkie-ai/chonkie