| 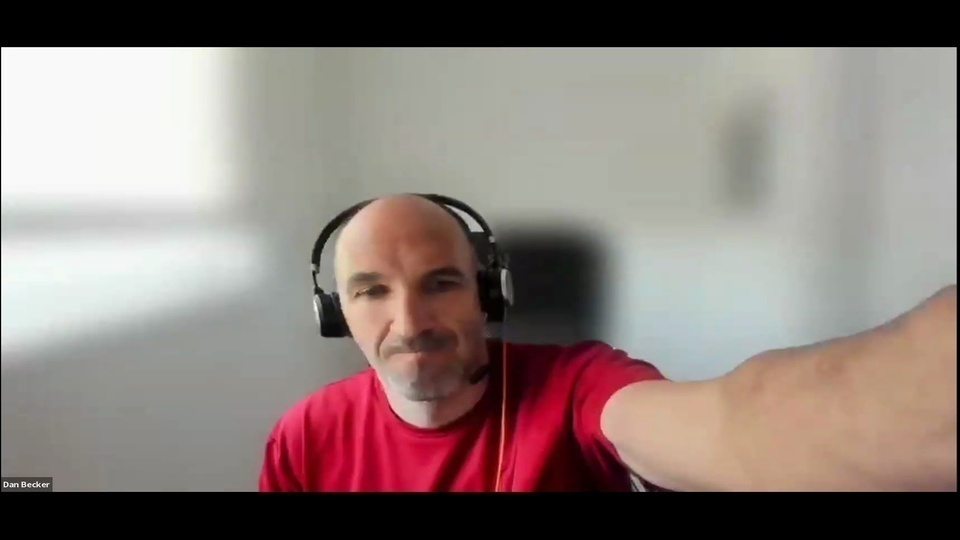 圖片編號: 1 圖片時間: 0.00秒 |
Dan Becker: Everyone. Dan Becker: the Joe Dan Becker: to him. Hamel Husain: Hello! Jo: Hello! Hamel Husain: Really excited about this talk is also the link. Jo: Yeah. Hamel Husain: Last one of the conference. Hamel Husain: So it's very special. So. Jo: Thank you so much for inviting me. Hamel Husain: Yes. Jo: Fantastic. Yeah, it's amazing to see all the interest in your course. And I mean the lineup of Speaker. So I was really honored when you. Jo: when you ask me to to join. That's amazing. Hamel Husain: No, I'm really honored to have you to have you join. Hamel Husain: So yeah, it's great. Hamel Husain: Think your perspectives on on rag are are very good. Dan Becker:大家好。 Dan Becker:Joe。 Dan Becker:給他。 Hamel Husain:你好! Jo:你好! Hamel Husain:真的很期待這次的演講,這裡也有連結。 Jo:是的。 Hamel Husain:這是會議的最後一場演講。 Hamel Husain:所以非常特別。 Jo:非常感謝你邀請我。 Hamel Husain:是的。 Jo:太棒了。是的,看到大家對你的課程這麼感興趣,真的很驚人。而且演講者的陣容也很強大。所以當你邀請我加入時,我真的很榮幸。 Jo:當你邀請我加入時,真的很驚人。 Hamel Husain:不,我真的很榮幸你能加入。 Hamel Husain:是的,這太好了。 Hamel Husain:我認為你對 RAG 的觀點非常好。 | 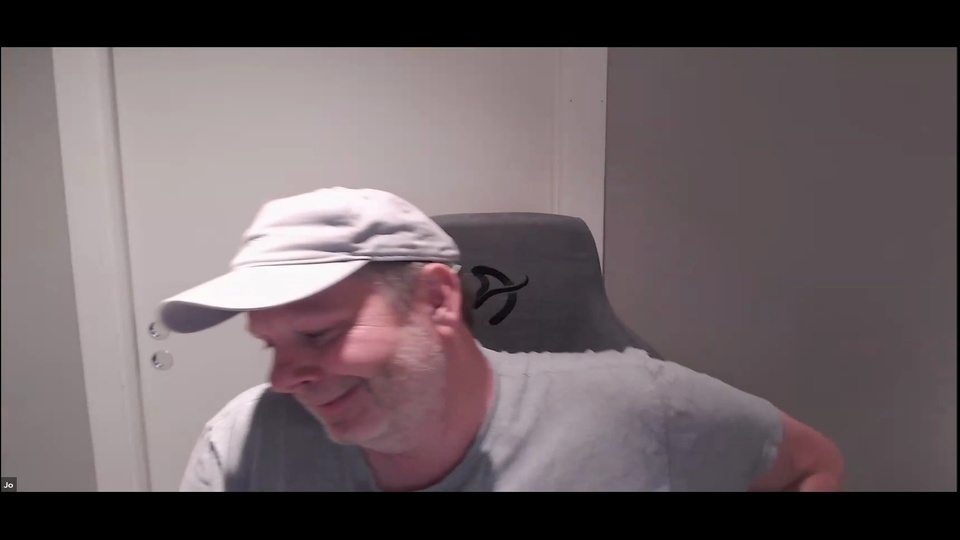 圖片編號: 16 圖片時間: 75.00秒 |
Hamel Husain: so I'm excited about you sharing it more widely. Jo: Thank you. Dan Becker: We usually Dan Becker: start like 5 after the hour or after the hour. So Dan Becker: got another 5 min. Dan Becker: we have Dan Becker: 23 people who are watching or listening to us now. Dan Becker: I bet we'll end up probably Dan Becker: hard to say with this being the last one. But I'm guessing we'll end up, probably around a hundred Dan Becker: map. Jo: Sounds, great. Hamel Husain: Trying to think. Is there a Hamel Husain: the score channel for this talk? Dan Becker: Yeah, I just made one. Dan Becker: So it is Dan Becker: posted a link in bergamag. Dan Becker: and I just posted a link to it in general. Hamel Husain: They don't sort it alphabetically, alphabetically. They were sorted by Hamel Husain: some kind of Hamel Husain: Okay, see you here. Dan Becker: Could be chronologically based on when it was created. I was thinking about the same thing. Hamel Husain:所以我很高興你能更廣泛地分享這個內容。 Jo:謝謝。 Dan Becker:我們通常 Dan Becker:在整點或過了五分鐘開始。所以 Dan Becker:還有五分鐘。 Dan Becker:我們現在有 Dan Becker:23個人在觀看或聆聽我們的講話。 Dan Becker:我打賭我們最終可能會 Dan Becker:很難說,因為這是最後一次。但我猜我們最終可能會有大約一百人 Dan Becker:地圖。 Jo:聽起來不錯。 Hamel Husain:試著想想。這次講座有 Hamel Husain:專門的頻道嗎? Dan Becker:是的,我剛剛創建了一個。 Dan Becker:所以它是 Dan Becker:在 bergamag 上發布了一個鏈接。 Dan Becker:我剛剛在 general 頻道也發布了一個鏈接。 Hamel Husain:他們不是按字母順序排序的。他們是按 Hamel Husain:某種 Hamel Husain:好的,見到你了。 Dan Becker:可能是按創建時間排序的。我也在想同樣的事情。 | 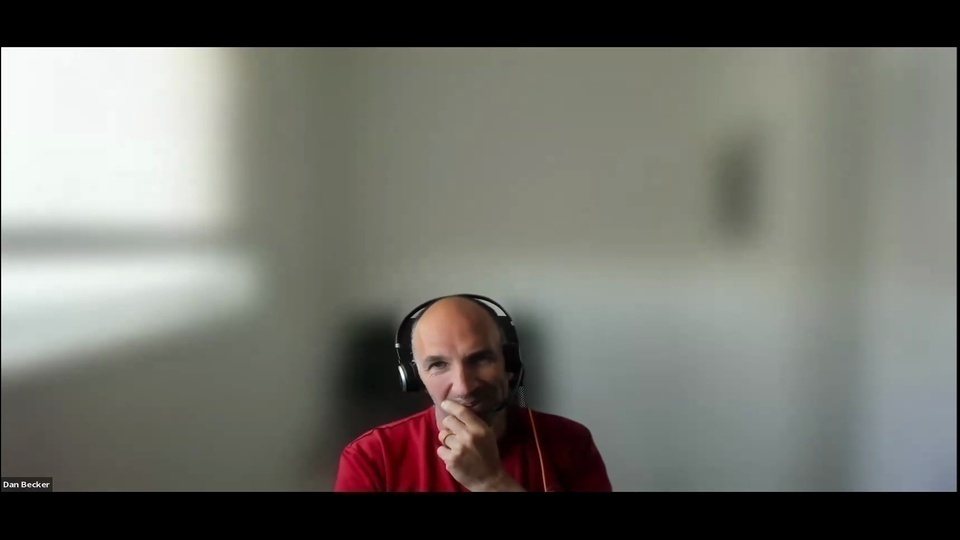 圖片編號: 31 圖片時間: 150.00秒 |
Dan Becker: That's not bad. Well, who even knows? Dan Becker: Early on, Joe? I asked. You know, in these 5 min, when some people are waiting Dan Becker: what they want to hear us talk about. Dan Becker: And they the popular response was war stories. So either war stories or what? Something Dan Becker: that in your coding Dan Becker: has not worked or gone wrong Dan Becker: in the last week. Jo: In the last week. Jo: Hmm. Jo: no, I I yeah, I don't have a lot of war stories for this week. But Jo: I've been trying out some new techniques for evaluating such results. So I'll share some some of those results in this in this talk. Yeah. So you you make some interesting findings. And then you also do some mistakes. Use Co pilot a lot and it's auto completions are basically Dan Becker:那還不錯。嗯,誰知道呢?
Dan Becker:Joe,早些時候?我問過。在這 5 分鐘內,有些人在等待的時候
Dan Becker:他們想聽我們談論什麼。
Dan Becker:而他們最受歡迎的回應是戰爭故事。所以要麼是戰爭故事,要麼是什麼?某些
Dan Becker:在你的編碼中
Dan Becker:在過去一週內沒有成功或出錯的事情。
Dan Becker:在過去一週內。
Jo:在過去一週內。
Jo:嗯。
Jo:不,我,我是的,我這週沒有很多戰爭故事。但是
Jo:我一直在嘗試一些新的技術來評估這些結果。所以我會在這次演講中分享一些這些結果。是的。所以你會有一些有趣的發現。然後你也會犯一些錯誤。經常使用 Co pilot,它的自動完成基本上是 | 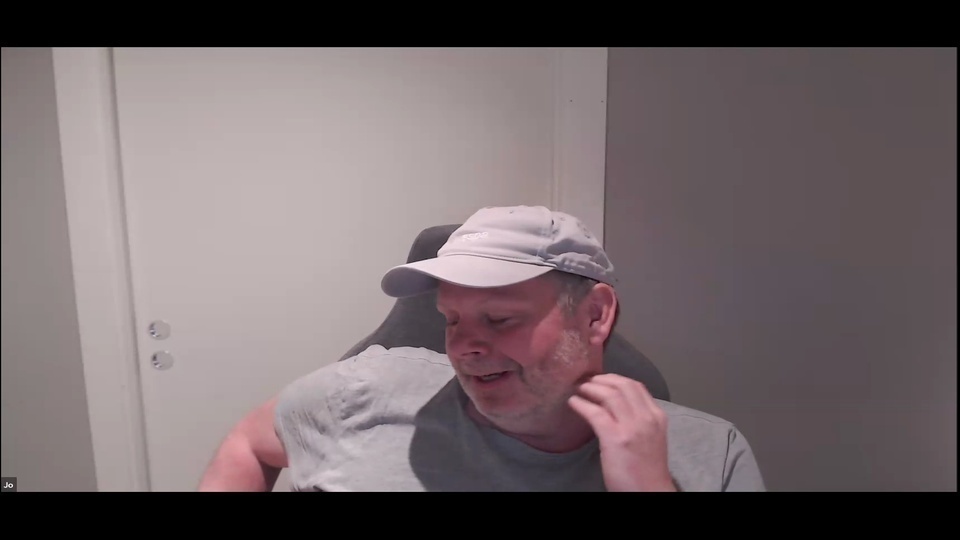 圖片編號: 47 圖片時間: 230.00秒 |
Jo: a couple of years ago, some, the Openai Apis are changing. And so, yeah. Jo: not that interesting though. Dan Becker: Yeah, you know, with copilot Dan Becker: W with rag, you do a lot of metadata filtering so that you try and get more recent results. And it feels to me that with large language models more broadly. Dan Becker: it'd be nice to do something so that it tries to auto complete with newer results rather than older ones you could imagine. Like, when you calculate loss functions. Dan Becker: there's a weight involved in that weight as a function of when the training data is from or it'd be nice if there was something like that. Dan Becker: But Jo: Yeah, it was also interesting from. Jo: I think, Jo: the existing technologies like Xql. Databases. The completions are pretty good, both from shutt, Gpt and and general language models because they have a good. Jo: It's a lot of that data in their training data, basically. But Jo: if you have a new product with some new Apis. The cold completions Jo:幾年前,Openai 的 API 發生了一些變化。所以,是的。 Jo:不過,這並不是那麼有趣。 Dan Becker:是的,你知道,使用 copilot Dan Becker:使用 RAG,你會做很多元數據過濾,這樣你可以嘗試獲得更近期的結果。對我來說,這感覺像是使用更廣泛的 LLM。 Dan Becker:如果能做些什麼,讓它嘗試自動完成更新的結果而不是舊的結果,那就太好了。你可以想像一下,當你計算損失函數時。 Dan Becker:這其中涉及到一個權重,這個權重是訓練數據的時間函數。如果有類似的東西,那就太好了。 Dan Becker:但是 Jo:是的,這也很有趣。 Jo:我認為, Jo:現有的技術如 Xql 數據庫。無論是 shutt、Gpt 還是一般的語言模型,完成度都相當不錯,因為它們的訓練數據中有很多這樣的數據。基本上是這樣的。 Jo:但如果你有一個帶有新 API 的新產品。冷啟動完成度 | 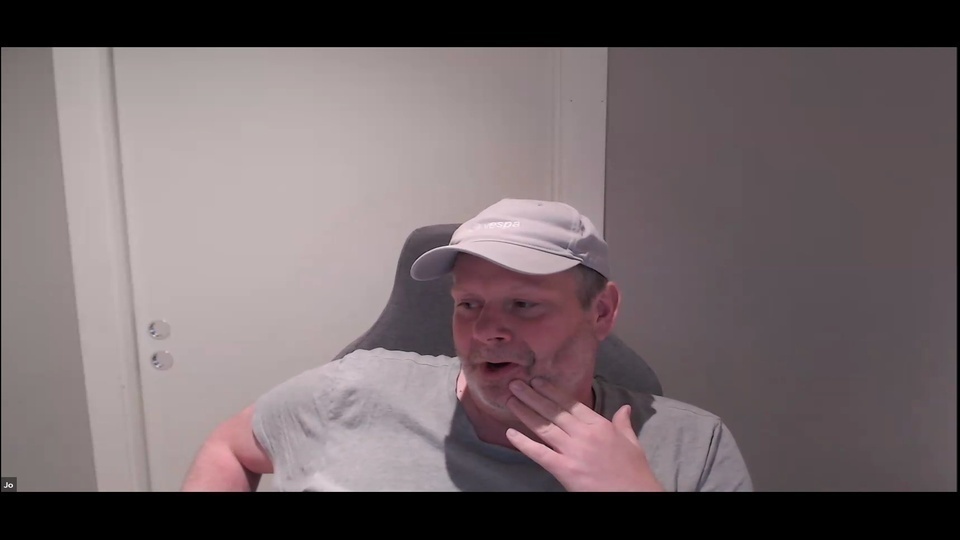 圖片編號: 63 圖片時間: 310.00秒 |
Jo: don't work that. Well. So that's why we at last. But we also try to Jo: build our own rag solution on on search, less by AI to, you know. Help people use use Vespa and that's 1 of the things that been frustrating with these language models is that they are Jo: quite familiar with elastic because elastic search has been around for Jo: quite some time. But West place is is newer in the public domain. So Jo: people are getting better completions for elastic search than than less. Also. Jo: we have to do something about that. Dan Becker: Damp. Jo: Yeah, I see some great questions already. So that's fantastic. So I'm I'm I'm planning on. Jo:這樣不行。所以這就是為什麼我們最後。但是我們也嘗試 Jo:在搜索上構建我們自己的 RAG 解決方案,少用 AI,你知道的。幫助人們使用 Vespa,這是這些語言模型令人沮喪的地方之一,就是它們 Jo:對 Elastic 相當熟悉,因為 Elastic Search 已經存在 Jo:相當長的一段時間了。但是 Vespa 在公共領域是比較新的。所以 Jo:人們對 Elastic Search 的完成度比 Vespa 更好。所以。 Jo:我們必須做些什麼來改變這種情況。 Dan Becker:潮濕。 Jo:是的,我已經看到一些很棒的問題了。所以這太棒了。所以我我我計劃。 | 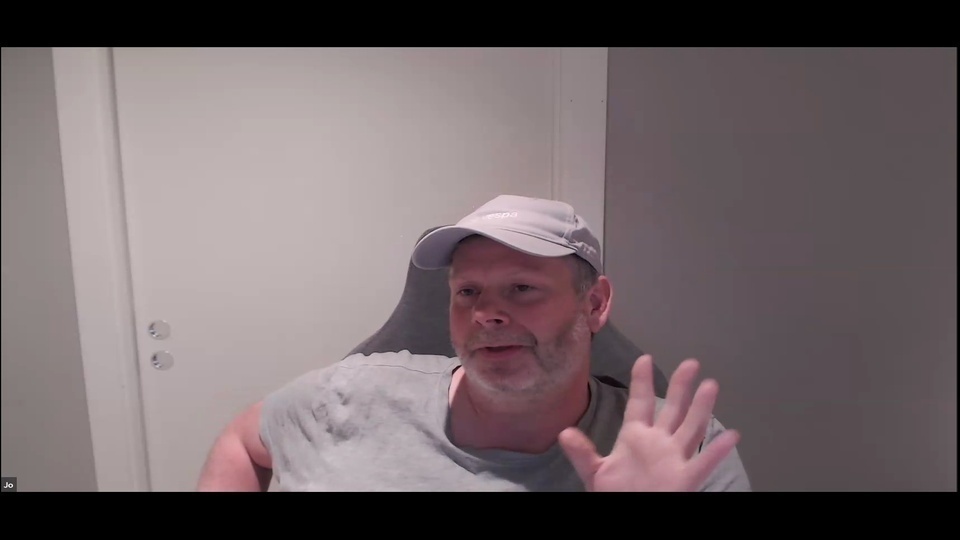 圖片編號: 78 圖片時間: 385.00秒 |
Jo: I'm not sure how much time, because there were quite a few invites, but I'm hoping to spend a half an hour talking. Jo: and that we could have an open session. So you know, drop your questions that that's that's awesome. Jo: So we can get the discussion going. Jo: And there's there. Yeah, there's a lot of things to be excited about in in in search, and I'll I'll cover some of them, and especially around evaluations. So so, major bulk in this talk will be about setting up your own evaluations so that you can actually Jo: make changes and iterate on on search and actually measuring Jo: the impact of of that Jo: and it doesn't need to be very fancy to have something that you can actually iterate on so Jo: and thankfully. Large language models can also help us there. Thanks to recent advances. So so I think that's that's interesting Jo: in it. Jo:我不確定有多少時間,因為有很多邀請,但我希望能花半小時來講話。 Jo:然後我們可以有一個開放的討論環節。所以你知道,提出你的問題,那真是太棒了。 Jo:這樣我們就可以開始討論了。 Jo:而且有很多值得興奮的事情,特別是在檢索方面,我會涵蓋其中的一些,尤其是關於評估的部分。所以,本次演講的主要部分將是關於設置你自己的評估,以便你能夠實際 Jo:進行變更和迭代檢索,並實際測量 Jo:這些變更的影響 Jo:而且不需要非常複雜的設置,你就可以進行迭代 Jo:幸運的是,得益於最近的進展,大型語言模型也可以在這方面幫助我們。所以我認為這很有趣。 Jo: | 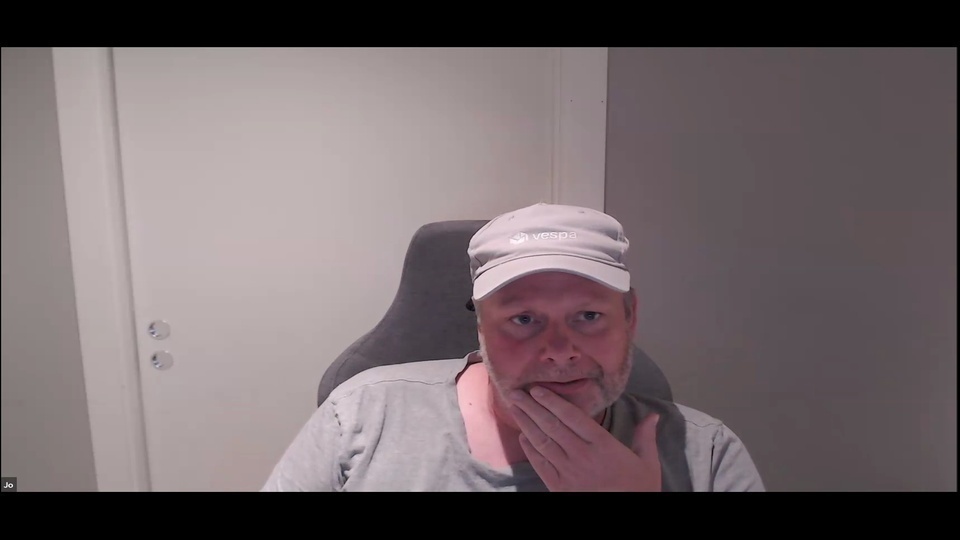 圖片編號: 93 圖片時間: 460.00秒 |
Jo: So I'll try to share my Jo:所以我會嘗試分享我的 | 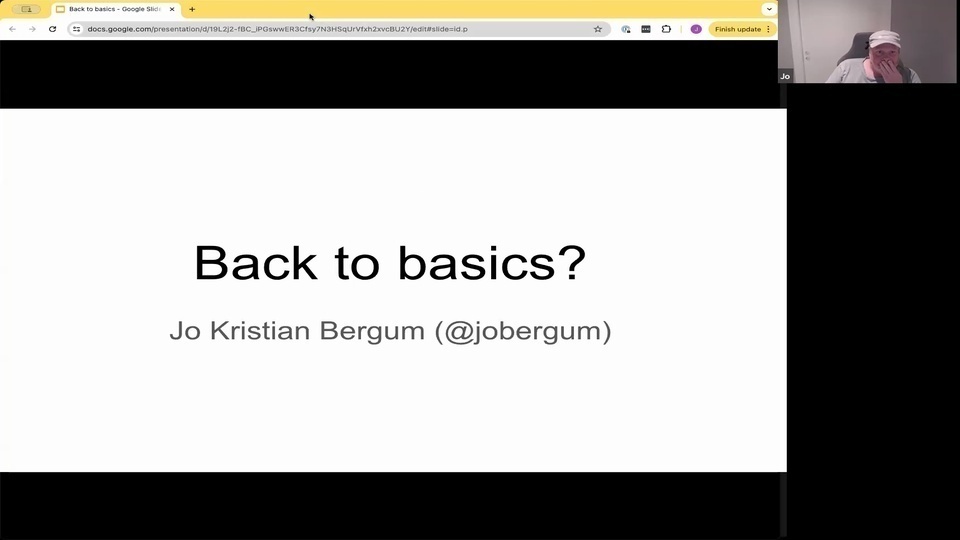 圖片編號: 94 圖片時間: 465.00秒 |
Jo: presentation, see if everything is Jo: working well. Dan Becker: We can see it. Jo: You see it. Okay. Jo: zoom is so much better than meat. Hamel Husain: Yeah, I agree with that. Dan Becker: Yeah. Dan Becker: it's I guess Google has fortunately bridge at 1 point. Yeah, they have, like 10 different solutions for. Jo: Yeah, yeah. Dan Becker: I think they they've probably consolidated them, but Dan Becker: they haven't used that to make them dramatically better. Jo: Yeah, because in meet, when you run percent Jo: like, everything just disappears. You're like, okay, here, I have the full view. Jo: Yeah. Jo: that's an improvement. Dan Becker: Alright! You know, we're 5 after. We got about 100 people. Dan Becker: do you? Wanna wait another minute or 2. That's great. But otherwise I think you can start anytime. Jo: Yeah, sure, I can Jo: just get started. Yeah. So thank you for having me. I'll talk about back to basics. Jo: And I'm Joe Kristen Bergam. Jo: And let's see if I can. Actually, yeah. So about me. I'm a distinguished engineer. I work at west by AI, Jo:演講,看看一切是否 Jo:運作良好。 Dan Becker:我們可以看到。 Jo:你看到了。好的。 Jo:Zoom 比 Meet 好太多了。 Hamel Husain:是的,我同意。 Dan Becker:是的。 Dan Becker:我猜 Google 曾經在某個時候架起了橋樑。是的,他們有大約 10 種不同的解決方案。 Jo:是的,是的。 Dan Becker:我想他們可能已經整合了它們,但 Dan Becker:他們並沒有利用這一點來使它們顯著變得更好。 Jo:是的,因為在 Meet 中,當你運行百分比時 Jo:就像一切都消失了。你會想,好吧,在這裡,我有完整的視圖。 Jo:是的。 Jo:這是一個改進。 Dan Becker:好的!你知道,我們已經過了 5 分鐘。我們大約有 100 人。 Dan Becker:你想再等一兩分鐘嗎?那很好。但否則我認為你可以隨時開始。 Jo:是的,當然,我可以 Jo:直接開始。是的。所以謝謝你邀請我。我會談談回歸基礎。 Jo:我是 Joe Kristen Bergam。 Jo:讓我們看看我是否可以。事實上,是的。關於我。我是一名傑出的工程師。我在 West by AI 工作。 | 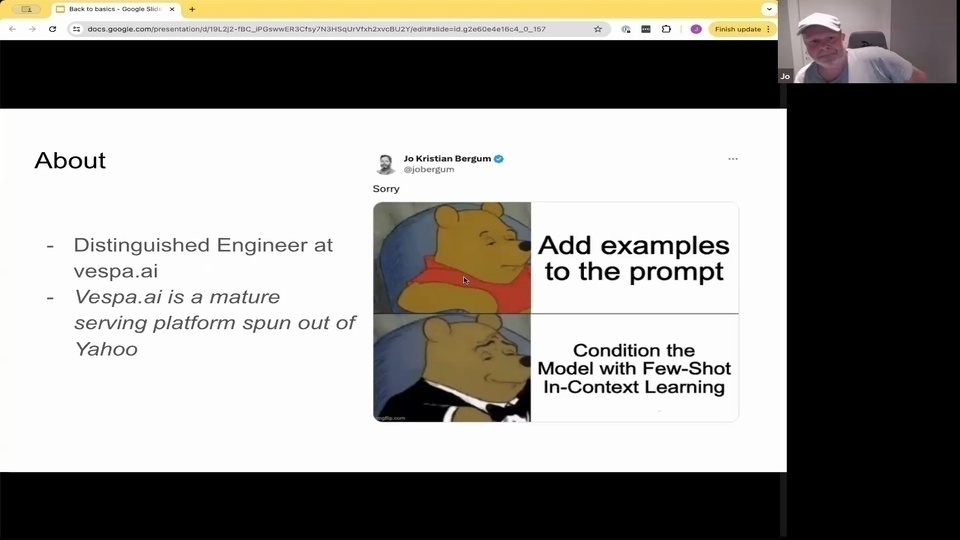 圖片編號: 111 圖片時間: 550.00秒 |
Jo: and I've been at best by AI for 18 years. Actually. So, I've been working in search and recommendation space for about 20 years and best by eyes, basically a platform serving platform that was recently spun out of Yahoo. We've been open source since 2,017, and in my spare time I spend some time on twitter posting memes. Jo: yeah. And in this talk I'll talk about stuffing text into the language model. Prompt. I'll talk about information, retrieval. Jo: The r and rag. Jo: And most of the talk will be about evaluation of these systems of information with digital systems Jo:我在 Best by AI 工作了 18 年。其實,我在搜索和推薦領域工作了大約 20 年,而 Best by AI 基本上是一個最近從 Yahoo 分拆出來的平台服務平台。我們從 2017 年開始開源,在空閒時間,我會在 Twitter 上發佈一些迷因。
Jo:是的,在這次演講中,我會談論如何將文本填充到語言模型中。我會談論信息檢索。
Jo:R 和 RAG。
Jo:大部分演講將圍繞這些數字系統的信息評估。 | 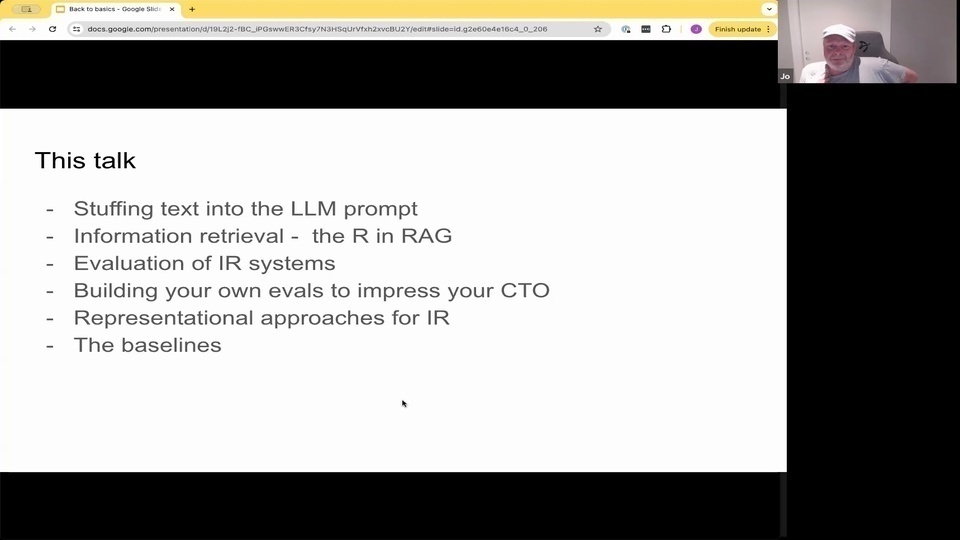 圖片編號: 121 圖片時間: 600.00秒 |
Jo: and how you can build your own emails to impress your CTO. Jo: And I'll talk about representational approaches for information. Retrieval. This includes Pm. 25 vectors, embeddings, whatnot. Jo: and some of the baselines. Jo: So rag. Jo: You're all familiar with Rag, but I think it's also interesting that you can use the kind of the whole rag concept to Jo:以及如何構建自己的電子郵件來打動你的 CTO。 Jo:我將討論信息檢索的表示方法。這包括 Pm. 25 向量、嵌入等。 Jo:以及一些基線。 Jo:所以 RAG。 Jo:你們都熟悉 RAG,但我認為使用整個 RAG 概念也很有趣。 | 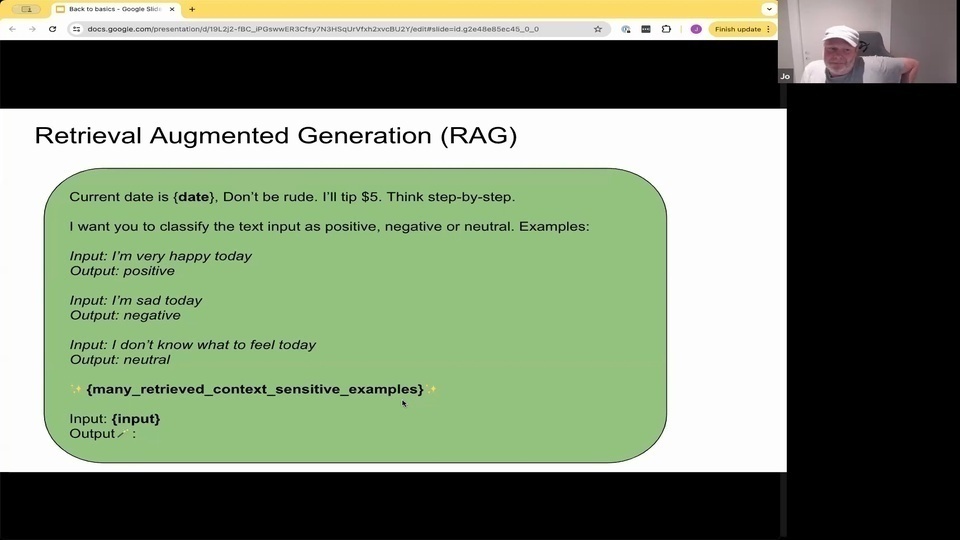 圖片編號: 126 圖片時間: 625.00秒 |
Jo: stuff Jo: things into the prompt, not necessarily related to question answering or search. Jo: But, for example, if we are building a labeler or a classifier, we can also use retrieval to retrieve kind of relevant examples or examples out of our training data sets right? Jo: So that's 1 way. But it's not that often discussed that you can also use retrieval. So let's say, you have 1 billion annotated training examples. You can actually use retrieval to retrieve relevant Jo: examples and then have the large language models. Reason around that and Jo: predict a label. Jo:將東西放入提示中,不一定與問答或搜索有關。
Jo:但是,例如,如果我們正在構建一個標籤器或分類器,我們也可以使用檢索來檢索相關的示例或從我們的訓練數據集中檢索示例,對吧?
Jo:這是一種方法。但很少討論到你也可以使用檢索。所以假設你有 10 億個註釋的訓練示例。你實際上可以使用檢索來檢索相關的
Jo:示例,然後讓大型語言模型圍繞這些示例進行推理並
Jo:預測標籤。 | 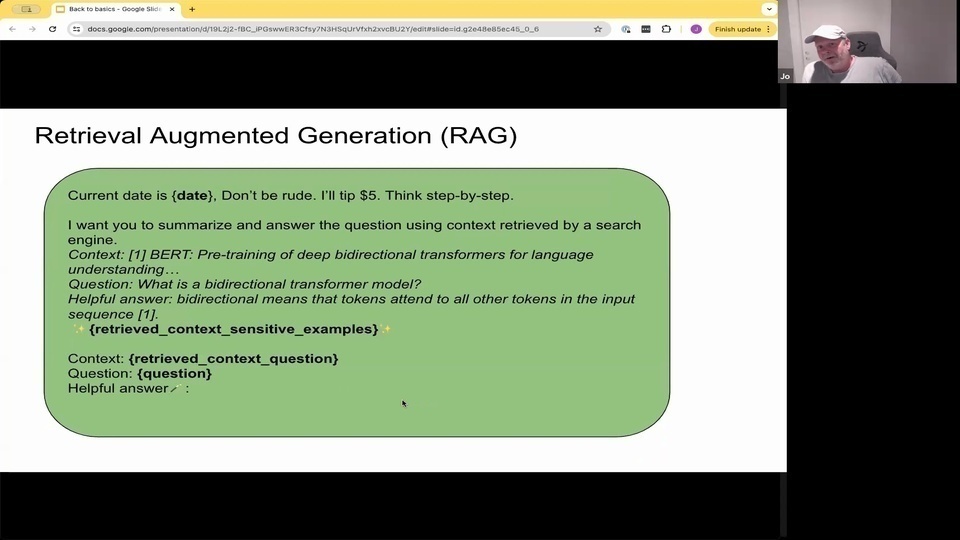 圖片編號: 135 圖片時間: 670.00秒 |
Jo: But most are Jo: thinking about rag in the context of building this kind of Jo: question answering model that you see at Google, and all these chat bots and and similar, where you retrieve for a question open ended question. And then you retrieve some hopefully relevant context. And you then stuff that into the prompt. And then you have Jo: hopefully, the language model will generate. A grounded response. It might not be how this nation free. But some say that it improves the kind of accuracy of the generation stuff. Jo:但大多數人 Jo:在思考 RAG 時,都是在構建這種類型的 Jo:問答模型,就像你在 Google 和所有這些聊天機器人中看到的一樣,對於一個開放式問題進行檢索。然後你檢索一些希望相關的上下文。然後你將其填入提示中。然後你有 Jo:希望語言模型會生成一個有根據的回應。這可能不是這個國家的自由。但有些人說這提高了生成內容的準確性。 | 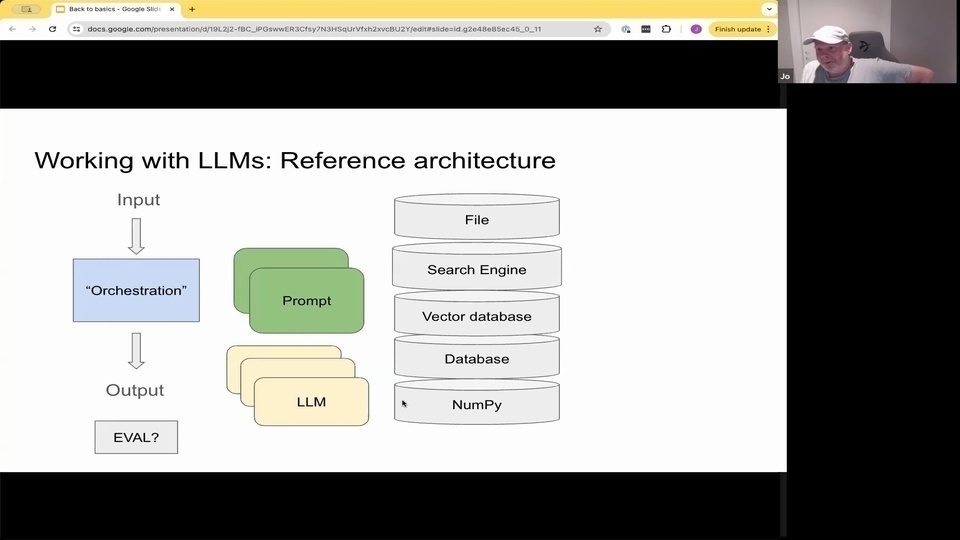 圖片編號: 144 圖片時間: 715.00秒 |
Jo: So Jo: that's kind of demystifying it. Jo: And working with these like reference architecture. There's some orchestration component. There's some input, there's some output. Hopefully, you have some evaluation of that output prompting different language models. And then you have kind of state which can be files, search engines vector, databases, regular databases, or even numpy Jo: so there's a lot of things going on there. Jo:所以
Jo:這有點解密的感覺。
Jo:並且與這些參考架構一起工作。有一些編排組件。有一些輸入,有一些輸出。希望你能對這些輸出進行評估,提示不同的語言模型。然後你會有一些狀態,這些狀態可以是文件、搜索引擎向量、數據庫、常規數據庫,甚至是 numpy
Jo:所以這裡有很多事情在進行。 | 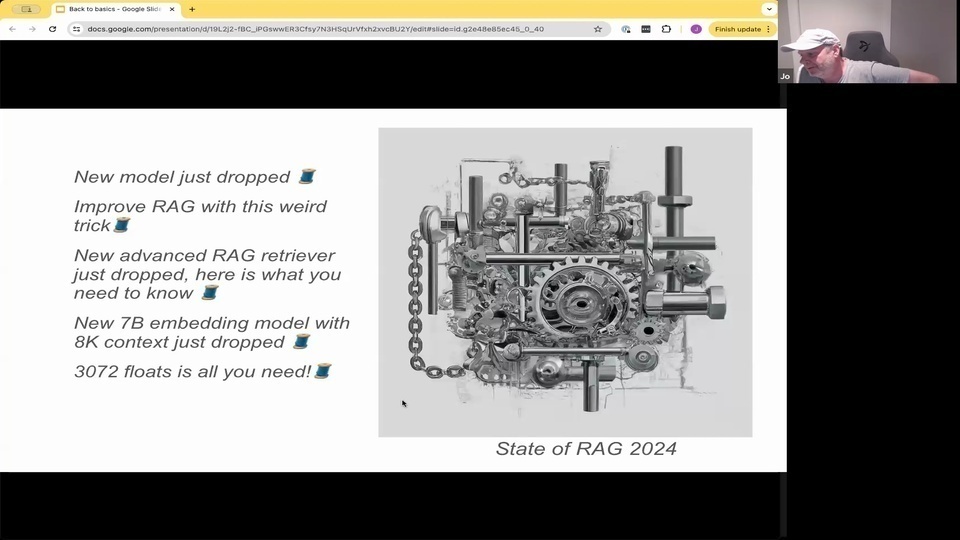 圖片編號: 150 圖片時間: 745.00秒 |
Jo: and Jo: there's a lot of hype around rag and also different methods for doing rag. And I'm on Twitter a lot. And I see all this kind of Jo: Twitter. Trans, there's new model. So there's a lot of Jo: components in this machinery. Lots of new tricks check out this. So it's a lot of kind of hype. Jo: So I, I like to kind of try to cut through that. And you know what, what's what's behind this? How does this work on your data. This is actually, is this actually a model that actually have some basics or backing from research? Jo: Have you actually evaluated it on some data set. Jo: And I think Jo: this is it can be if you're like coming into this space. And you're new to retrieval. You're new to search, and you're new to language models. And you want to build something. There's a lot of Jo: confusing information going around. Jo:而且 Jo:關於 RAG 有很多炒作,也有不同的方法來實現 RAG。我經常在 Twitter 上看到這些東西。 Jo:Twitter 上有新的模型。所以這個機制中有很多組件。很多新技巧,看看這個。所以這有很多炒作。 Jo:所以我,我喜歡嘗試去除這些炒作。你知道,這背後是什麼?這在你的數據上是如何工作的。這實際上是一個有一些基礎或研究支持的模型嗎? Jo:你實際上有在某些數據集上評估過它嗎? Jo:而且我認為 Jo:如果你剛進入這個領域,你對檢索、搜索和語言模型都不熟悉,並且你想構建一些東西,這可能會讓你感到困惑。 Jo:有很多混亂的信息在流傳。 | 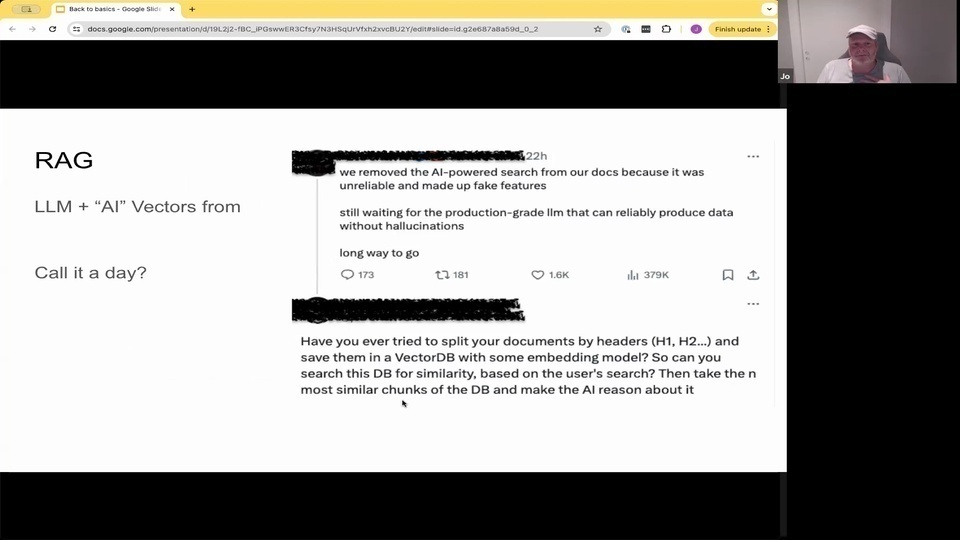 圖片編號: 161 圖片時間: 800.00秒 |
Jo: and I just saw this twitter thread about the rag, and people are losing faith in it. And you know we we removed AI powered search. Jo: And I think there's been like Jo: brag is only about Jo: taking a language model from, for example, Openai, and then they use their embeddings. And then you have a magical search experience, and that's all you need, and I think that's Jo: naive. To think that you can build a great product or a great rag solution in in that way, just by using vector embeddings and and the language models. Jo: because there are the retrieval stack in this pipeline the process of obtaining relevant information based on some query basically has been around, for, like Benjamin and his talk covered for decades. And Jo: there are a lot of people or the brightest minds that I've actually spent a lot of time on on retrieval and search right? Jo: Because it's so relevant across many kind of multi 1 billion companies like recommendation services search like Google Bing and whatnot. So this is a kind of always been a very hot and interesting topic. Jo:我剛剛看到這個關於 RAG 的 Twitter 討論串,人們正在對它失去信心。你知道我們移除了 AI 驅動的搜索。
Jo:我認為這裡有一點
Jo:吹噓的只是
Jo:從例如 OpenAI 取得一個語言模型,然後他們使用他們的嵌入。然後你就有了一個神奇的搜索體驗,這就是你所需要的,我認為這
Jo:太天真了。認為你可以僅僅通過使用向量嵌入和語言模型來構建一個偉大的產品或一個偉大的 RAG 解決方案。
Jo:因為在這個管道中有檢索堆棧,根據某些查詢獲取相關信息的過程基本上已經存在了幾十年,正如 Benjamin 在他的演講中所涵蓋的那樣。
Jo:有很多人或最聰明的頭腦實際上花了很多時間在檢索和搜索上,對吧?
Jo:因為它在許多數十億美元的公司中都非常相關,比如推薦服務、搜索像 Google、Bing 等等。所以這一直是一個非常熱門和有趣的話題。 | 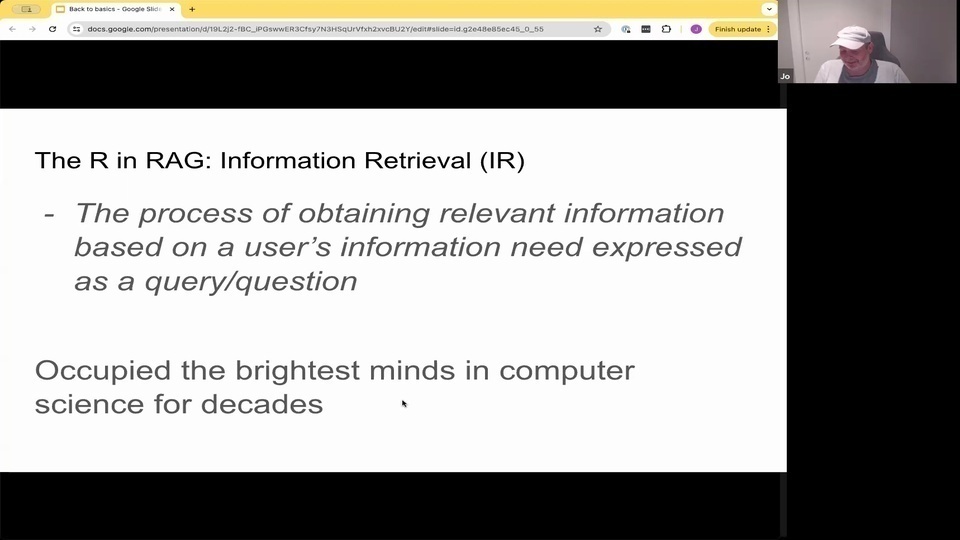 圖片編號: 175 圖片時間: 870.00秒 |
Jo: And it's much deeper than Jo: encoding your text into one vector, representation. And then that's it. Jo: But I'll talk about how we can emulate these information retrieval systems. And this kind of basically, you could treat this as a more or less of a green box Jo:而且這比將你的文本編碼成一個向量表示要深得多,然後就結束了。
Jo:但我會談論我們如何模擬這些信息檢索系統。基本上,你可以把這看作是一個或多或少的綠盒子。 | 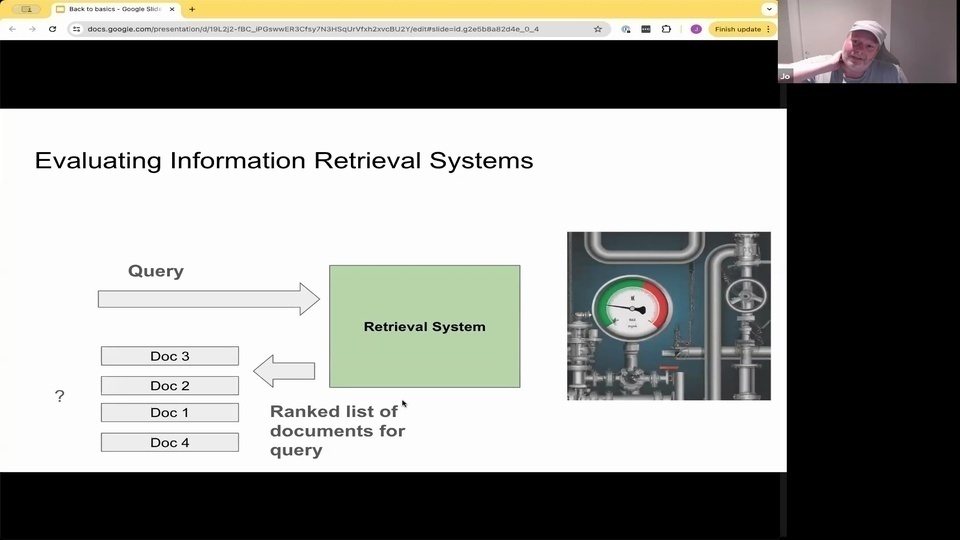 圖片編號: 179 圖片時間: 890.00秒 |
Jo: where you have put some data into late, and you have your kind of retrieval system. And you're asking that retrieval system, a question, and you're getting back a ranked list of documents. Jo: And then you can evaluate these documents and the quality of these documents. With regards to relevance of of how relevant they are. With regards to the query. Jo: And this is kind of independent. If you're using what kind of retrieval method you're using, or combination, or hybrids, or face ranking or culvert, or splayed, or whatnot you can evaluate any type of system if it's using numpy or files or or whatnot, it doesn't really matter. Jo:你已經輸入了一些數據,並且有一個檢索系統。你向這個檢索系統提出一個問題,然後你會得到一個排序的文檔列表。 Jo:然後你可以評估這些文檔及其質量,看看它們與查詢的相關性如何。 Jo:這與你使用哪種檢索方法無關,不管是單一方法、組合方法、混合方法、面排名、ColBERT、Splayed 等等,你都可以評估任何類型的系統,不管它是使用 numpy 還是文件或其他什麼,這並不重要。 | 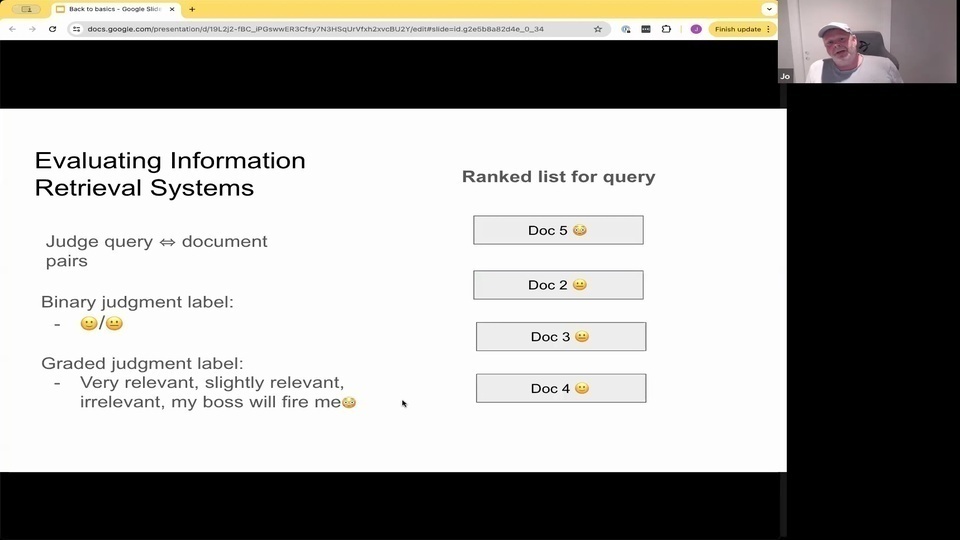 圖片編號: 189 圖片時間: 940.00秒 |
Jo: And the basic idea of this wiggling of such system is that you take a query and you retrieve those documents, and then you can have human annotator, for example. Jo: to judge the quality of each of the documents. And there are different ways of doing this. We can do it by the binary judgment, saying that, okay, this document is relevant for the query or not. Jo: or we can have a graded judgment where you say, okay, 0 means that the document is irrelevant for the query. And one, it's slightly relevant or 2 is highly relevant right? And we can also do use this to Jo: judge the rank lists that are coming out of recommendation systems or personalization and many different systems that are producing a rank list. Jo: And in information. Retrieval. This is going back decades. And there are a lot of researchers working on this. And you have track which is the text. Retrieval conference spans multiple different topics each year. News, retrieval, all kinds of different retrieval tasks. Ms. Marco. Maybe some of you are familiar with which is one of the largest data sets. That you can publish research on is from Bing, actually real Jo: data, which is annotated. And a lot of these embedding models are trained on this data set. Jo: Then we have beer from those rhymers at all that Jo: evaluate Jo: types of models without actually using the training data. But this is like in the 0 shot setting. So there are many different collections. And then there are metrics that can measure how well, the retrieval system is actually working. So we call at K, for example, K here, meaning a position in the in the ranking list, so K. Could be. For example, 10 or 20 or 100 or 1,000 Jo: and it's a metric that is focusing about. You know, you know, that there are like 6 items that are relevant for this query. And are we actually retrieving those 6 relevant documents Jo:這種系統的基本想法是,你提出一個查詢並檢索那些文件,然後你可以讓人工註解員來判斷每個文件的質量。有不同的方法可以做到這一點。我們可以通過二元判斷來做,比如說,這個文件對查詢是否相關。
Jo:或者我們可以有一個分級判斷,比如說,0 表示文件對查詢不相關,1 表示稍微相關,2 表示高度相關,對吧?我們也可以用這個來判斷推薦系統或個性化系統產生的排名列表,以及許多不同系統產生的排名列表。
Jo:在信息檢索中,這可以追溯到幾十年前。有很多研究人員在這方面工作。你有 TREC(文本檢索會議),每年涵蓋多個不同的主題,新聞檢索,各種不同的檢索任務。Ms. Marco,也許你們中的一些人熟悉,這是最大的數據集之一。你可以在上面發表研究,這實際上是來自 Bing 的真實數據,這些數據是經過註解的。很多嵌入模型都是在這個數據集上訓練的。
Jo:然後我們有 BEIR,這些研究者評估模型類型,而不實際使用訓練數據。但這是在零樣本設置中。所以有很多不同的集合。然後有一些指標可以衡量檢索系統的實際工作效果。我們稱之為 at K,例如,K 在這裡意味著排名列表中的位置,所以 K 可以是 10、20、100 或 1,000。
Jo:這是一個關注的指標。你知道,有 6 個項目與這個查詢相關。我們是否實際檢索到了這 6 個相關文件。 | 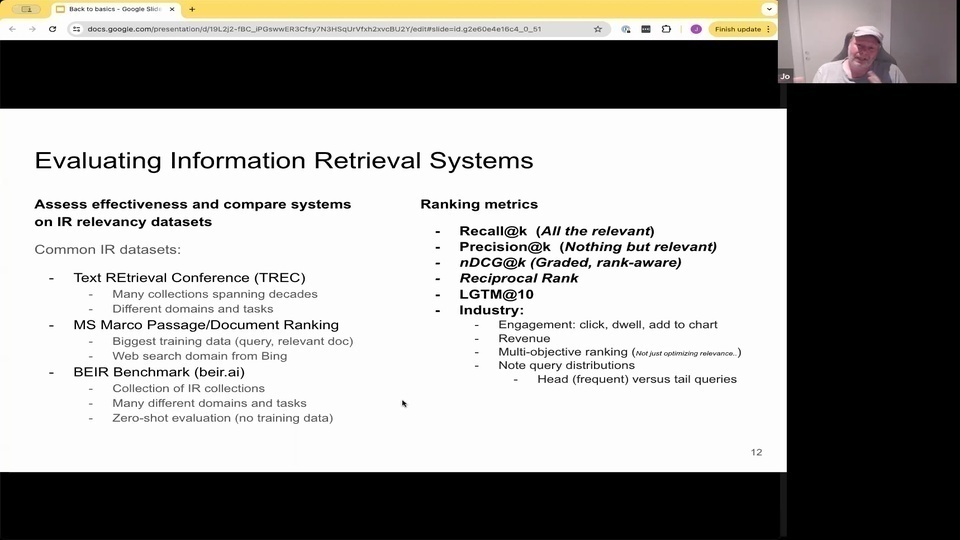 圖片編號: 215 圖片時間: 1070.00秒 |
Jo: into the to the top? K, in most systems, you don't actually know how many relevant documents there are in the collection in the web scale. It might be, you know, millions of documents that are relevant to the query, so it's not unless you have a really good control of your corpus. It's really difficult to kind of know. What are the actually relevant documents in in the document. But position is much easier because we can look at those results and say. Jo: Are there any irrelevant hits in in the top. K so precision is one. But it's not really rank aware. So it's not bothering. If the missing or relevant hit is placed at position one or 10, so the precision at 10 would be the same. If you. If it doesn't, it doesn't necessarily depend on the position. Jo: And DC d very complicated metric, but it tries to incorporate the labels so that the the graded labels, and also awareness of the rank position. If you wanna look up that you can. You could. Jo: You basically go to Wikipedia? But it's it's a quite advanced metric. Reciprocal rank measures. Where is the 1st relevant hit in the position. So if you place the relevant hit or a relevant hit at position one, you have a reciprocal rank of one. If you place the relevant hit at Position 2. You have a recipe rank of 0 point 5. Jo: Then, of course, you have Lgtm, which is, looks good to me, maybe the most common metric used in the industry. Jo: And of course, also in industry, you have other evaluation metrics like engagement. Jo: Click, if you're measuring what actually uses are interacting with the search dwell time or e-commerce. Add to chart all these kind of signals. That you can feed back. Jo: Of course, revenue e-commerce is search. For example, it's not only about the relevancy, but also you have some objectives for your business. Jo: I also like to point out that most of the benchmarks are comparing just a flat list, and then, when you're evaluating each of these queries, you get a score for each query, and then you take the average to kind of come up with a average number for the whole kind of retrieval method. Jo: But in practice in production systems, you will see that maybe 20% of the queries actually, is contributed like 80% of the volume. So you have to think a little bit about that when you're evaluating systems. Jo: Yeah, so. Jo: and to do better than looks good to me. Jo:進入頂端?K,在大多數系統中,你實際上不知道在網絡規模的集合中有多少相關文檔。可能有數百萬個與查詢相關的文檔,所以除非你對你的語料庫有非常好的控制,否則真的很難知道哪些文檔實際上是相關的。但位置要容易得多,因為我們可以查看這些結果並說。 Jo:在頂端 K 中是否有任何不相關的結果,所以精確度是一個指標。但它並不真正關注排名。所以如果缺失或相關的結果位於位置一或十,它並不在意,所以在十的位置的精確度會是相同的。如果你。如果它不依賴於位置。 Jo:而 DCG 是一個非常複雜的度量,但它試圖結合標籤,即分級標籤,並且也關注排名位置。如果你想查找,你可以。你可以去 Wikipedia,但這是一個相當高級的度量。倒數排名度量的是第一個相關結果的位置。所以如果你將相關結果或相關結果放在位置一,你的倒數排名是 1。如果你將相關結果放在位置二,你的倒數排名是 0.5。 Jo:當然,你還有 Lgtm,這看起來對我很好,可能是行業中最常用的度量。 Jo:當然,在行業中,你還有其他評估指標,比如互動。 Jo:點擊,如果你在測量實際用戶與搜索的互動時間或電子商務。添加到購物車所有這些信號。你可以反饋。 Jo:當然,電子商務的收入是搜索。例如,它不僅僅是關於相關性,還有你的業務目標。 Jo:我還想指出,大多數基準測試只是比較一個平面列表,然後當你評估每個查詢時,你會得到每個查詢的分數,然後你取平均值來得出整個檢索方法的平均數。 Jo:但在實際的生產系統中,你會看到可能 20% 的查詢實際上貢獻了 80% 的流量。所以在評估系統時,你需要考慮一下這一點。 Jo:是的,所以。 Jo:並且要做得比看起來對我好更好。 | 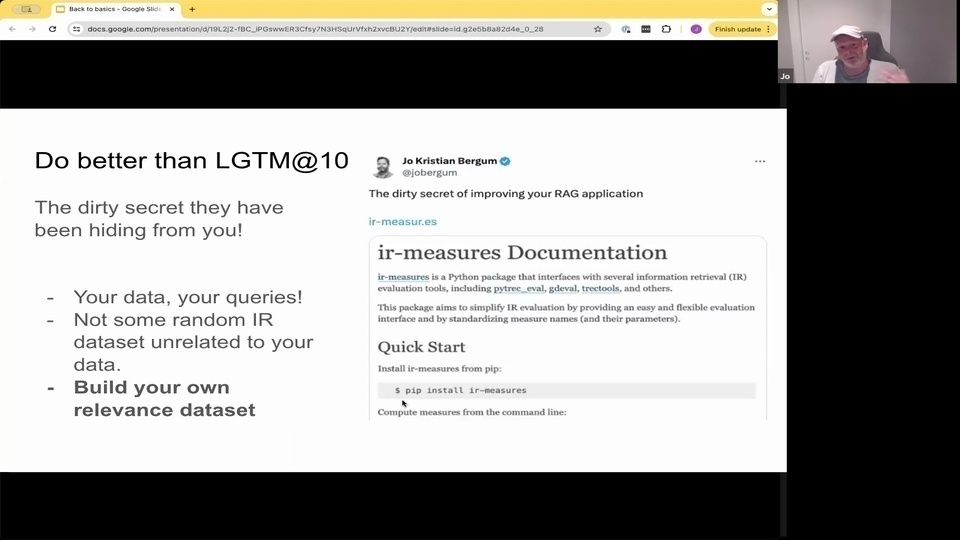 圖片編號: 249 圖片時間: 1240.00秒 |
Jo: you really have to measure. Jo: How you're doing. And Jo: since Jo: you have all these benchmarks, M. Tab and whatnot. Jo: they don't necessarily transfer to your domain or your use case. If you're building a rag application or retrieval application over code or documentation or specific health domain or products, or because there are different domains, different use cases. So your data, your queries. Jo: and the solution. To do better is to measure and building your own relevancy data set. Jo: And it's actually not that hard Jo: if you have actually a service in production. Jo: Look at what actually users are searching for and look at the results Jo: hand Jo: put in a few hours and judge the results. Jo: Might Jo: it actually relevant? Are you producing relevant results? And it doesn't really need to be fancy at all. And if you don't have traffic, if you haven't launched with it, you obviously have played around with the product. Jo:你真的必須進行測量。 Jo:你在做什麼。而且 Jo:因為 Jo:你有所有這些基準,M. Tab 之類的。 Jo:它們不一定能轉移到你的領域或你的使用案例。如果你正在構建一個 RAG 應用程序或檢索應用程序,無論是針對代碼、文檔、特定的健康領域或產品,因為有不同的領域,不同的使用案例。所以你的數據,你的查詢。 Jo:和解決方案。要做得更好就是測量並構建你自己的相關性數據集。 Jo:而且這其實並不難 Jo:如果你實際上有一個在生產中的服務。 Jo:看看用戶實際在搜索什麼,看看結果 Jo:手動 Jo:花幾個小時來判斷結果。 Jo:可能 Jo:它實際上相關嗎?你是否產生了相關的結果?而且這根本不需要花哨。如果你沒有流量,如果你還沒有推出它,你顯然已經玩過這個產品。 | 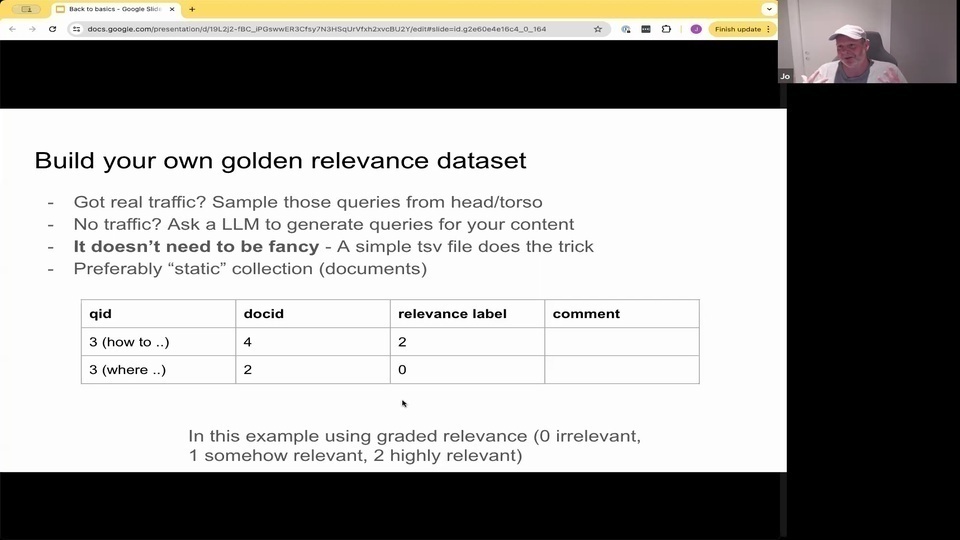 圖片編號: 262 圖片時間: 1305.00秒 |
Jo: or you can also ask a large language model to, you, know, present it some of your content, and then you can ask it, you know. Okay, what's the question that will be natural for a user to retrieve this kind of passage. So you kind of kind of Bootstrap even before you have any kind of user queries. Jo: And, as I said, it doesn't need to be fancy you can log. There are some fancy tools for doing this with user interface faces and docker and whatnot. But a simple Tsa Ts, tab separated file will do the trick. Preferably you will have like a static collection. But maybe not. Everybody has the luxury that you can actually have a static collection. And the reason why you would like to have a static collection is that Jo: when you are judging the results? Jo: And you're saying that for this query, for instance, query id 3. In the document. D. 5. You say that? Oh, this is a relevant one Jo: when we are judging the kind of, or computing the metric for the query, if there's a new document that is suddenly appearing, which is which is irrelevant or relevant. Jo: it might, you know, we might actually change thing in in the how we display things in in the ranking without we able to pick it up. So that's why you prefer. We have this kind of static collections. But and all the information retrieval data sets, they are usually static, right? They don't. They don't change. So we can evaluate methods and and practices over time. Jo: But Jo: you can also Jo: this process use language models to Jo: judge the results. And there's been interesting research coming out of Microsoft being team for over the last year, where they find that with some prompting techniques that they actually can have the large language models be Jo: pretty good at judging, query and and passages. So given a passage that this retreat for the query and they can ask, language model, is this relevant or not relevant? And they find that this actually correlates pretty well. And if you find, like a prompt combination that actually correlates with your data or your kind of golden data set, then you can start using this in at a more massive scale. Jo:或者你也可以請一個大型語言模型來,嗯,你知道,呈現一些你的內容,然後你可以問它,嗯,你知道。好,什麼問題對於用戶來說是自然的,可以檢索到這種段落。所以你可以在沒有任何用戶查詢之前就進行引導。
Jo:而且,正如我所說的,這不需要很花哨,你可以記錄。有一些花哨的工具可以用來做這個,有用戶界面和 docker 等等。但一個簡單的 Tsa Ts,制表符分隔文件就可以搞定。最好你會有一個靜態集合。但也許不是每個人都有這樣的奢侈,可以實際擁有一個靜態集合。你想要一個靜態集合的原因是
Jo:當你在評判結果的時候?
Jo:比如說,對於這個查詢,比如查詢 id 3。在文檔 D. 5 中。你說,哦,這是一個相關的
Jo:當我們在評判這種,或者計算查詢的指標時,如果突然出現一個新的文檔,這個文檔是無關的或相關的。
Jo:它可能,你知道,我們可能實際上會改變我們在排名中顯示事物的方式,而我們無法察覺。所以這就是為什麼你更喜歡我們有這種靜態集合。而且所有的信息檢索數據集,它們通常是靜態的,對吧?它們不會改變。所以我們可以隨著時間的推移評估方法和實踐。
Jo:但是
Jo:你也可以
Jo:這個過程使用語言模型來
Jo:評判結果。過去一年來,來自 Microsoft 團隊的一些有趣研究發現,通過一些提示技術,他們實際上可以讓大型語言模型在評判查詢和段落方面表現得相當不錯。所以給定一個查詢檢索到的段落,他們可以問語言模型,這是否相關。他們發現這實際上有很好的相關性。如果你找到一個與你的數據或你的黃金數據集相關的提示組合,那麼你可以開始在更大規模上使用這個方法。 | 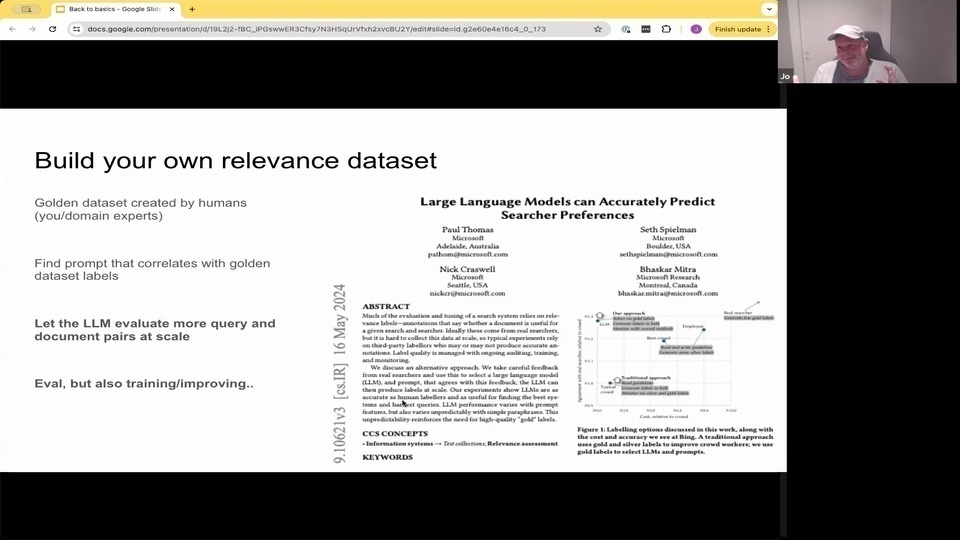 圖片編號: 289 圖片時間: 1440.00秒 |
Jo: and here's a very recent paper coming out 8 days ago where they also demonstrated that this prompt could actually work very well to assess the relevancy of the queries. Jo:『這裡有一篇非常近期的論文,8 天前發表的,他們也展示了這個提示實際上可以很好地評估查詢的相關性。』 | 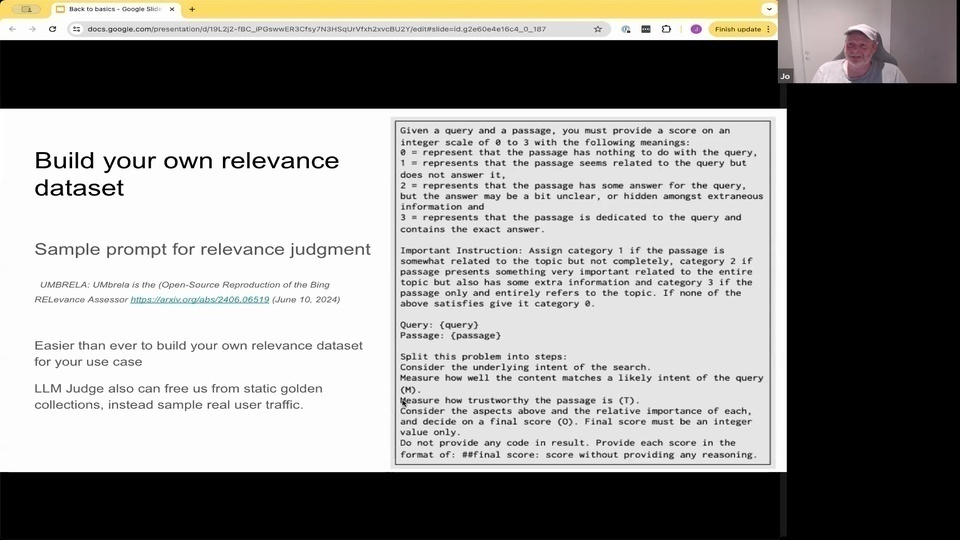 圖片編號: 294 圖片時間: 1465.00秒 |
Jo: And this can free us from this having this kind of static golden data set because we could start instead sampling real user queries and then ask the language model to evaluate the results. So I think this is a very interesting direction. Jo: And we have in our rag or vest by rag documentation search. I built like a small golden set with about 90 query passive judgments. Jo: and I just ran them through with this prompt or a similar prompt and I'm getting quite good correlation between what what I'm judging the results. And Gpt force is judging them, which is good because it means that I can now much cheaper judge, more results and then potentially also use this to adjust ranking. Jo:『這可以讓我們擺脫靜態的黃金數據集,因為我們可以開始抽取真實用戶查詢,然後讓語言模型來評估結果。所以我認為這是一個非常有趣的方向。』
Jo:『在我們的 RAG 或 vest by RAG 文件檢索中,我建立了一個小型的黃金集,大約有 90 個查詢被動判斷。』
Jo:『我只是用這個提示或類似的提示來運行它們,我發現我對結果的判斷和 GPT-4 的判斷之間有相當好的相關性,這很好,因為這意味著我現在可以用更低的成本來判斷更多的結果,然後也可以潛在地用這個來調整排名。』 | 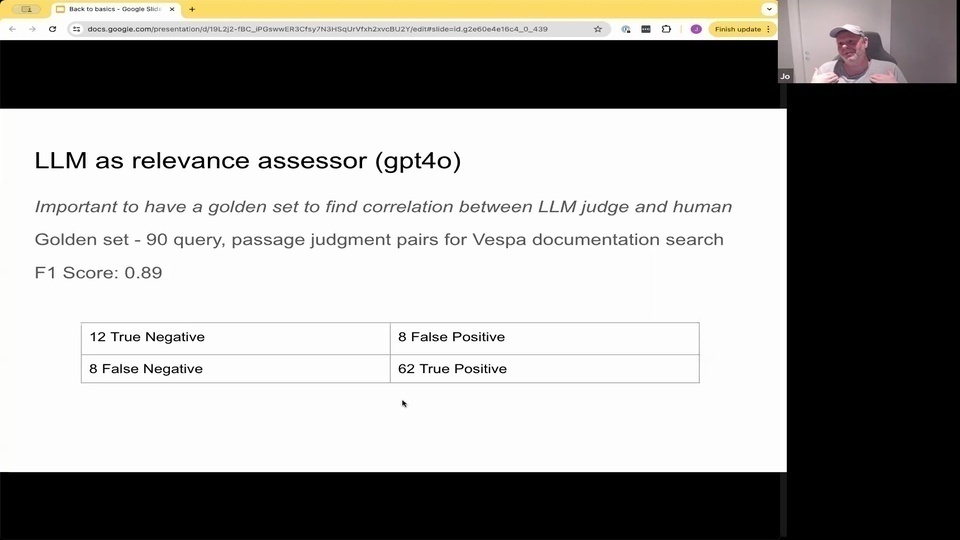 圖片編號: 302 圖片時間: 1505.00秒 |
Jo: Because when you have this kind of data set. Jo: you can also iterate and make changes. And then you can see, you know how it's actually performing. So instead of saying, Oh, we change something Jo: you can go to. We actually deploy this change that did increase. And Gtg, with 30%. And this is from Jo: our documentation vesper documentation search, which is relevant for us. It's our domain, you see here, Semantic, here is off the shelf vector embedding models. And then there are different ways in investment. To use. Hybrids won't go to the into the details. But now I actually have numbers on it. And then when I'm making changes. Jo:因為當你擁有這種數據集時,Jo:你也可以迭代並進行更改。然後你可以看到它實際上的表現。所以與其說,我們改變了某些東西,Jo:你可以說,我們實際部署了這個改變,並且提升了 30%。這是來自我們的文檔 vesper 文檔搜索,這對我們來說是相關的。這是我們的領域,你在這裡看到語義,這裡是現成的向量嵌入模型。然後在投資中有不同的使用方法。混合方法不會進入細節。但現在我實際上有數據了,然後當我進行更改時。 | 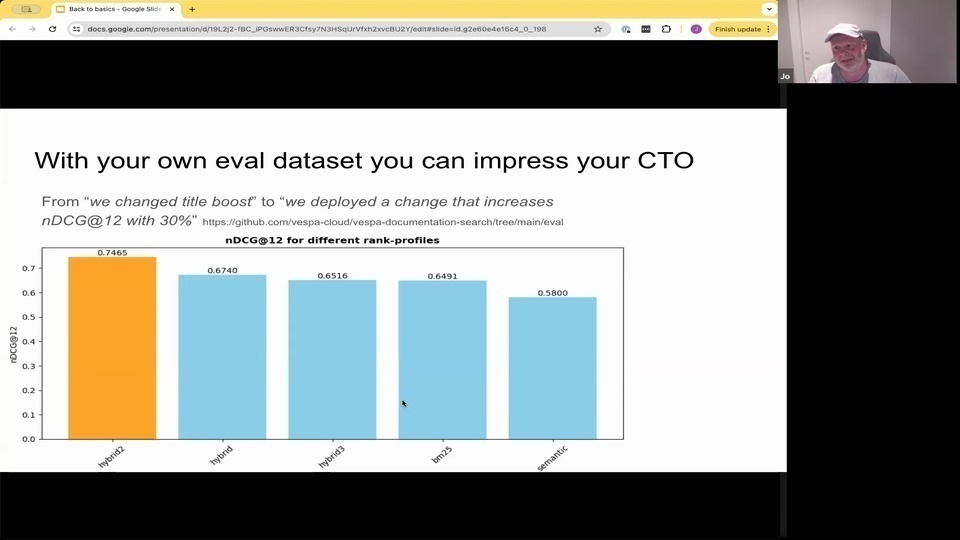 圖片編號: 311 圖片時間: 1550.00秒 |
| 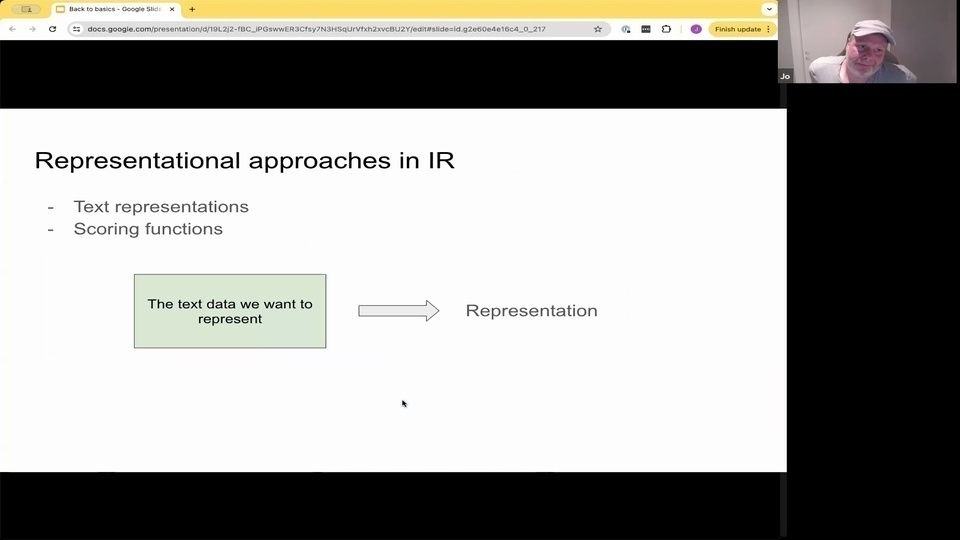 圖片編號: 315 圖片時間: 1570.00秒 |
Jo: So that's Jo: about evaluation so independent of the method or technique that we're using. Jo: we can evaluate the results coming out of the retrieval system. Jo: Now I want to talk a little bit about Jo: the representational approaches and and scoring functions that can be used for efficient retrieval. Jo:所以這是 Jo:關於評估,無論我們使用的方法或技術是什麼。 Jo:我們可以評估檢索系統的結果。 Jo:現在我想稍微談一下 Jo:可以用於高效檢索的表示方法和評分函數。 | 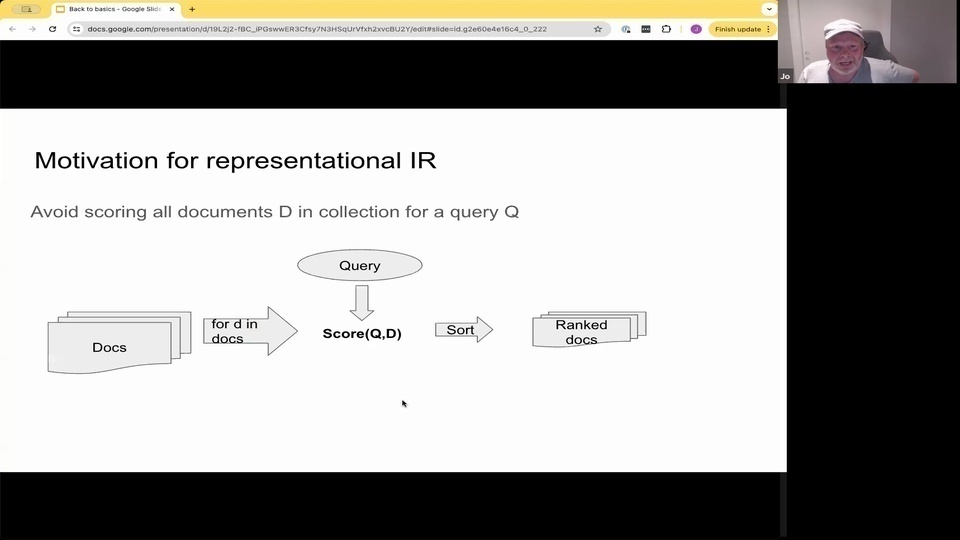 圖片編號: 319 圖片時間: 1590.00秒 |
Jo: and the motivation for having this kind of representational approach is that you want to try to avoid scoring all the documents in the collection. Jo: So if you're using, some of you might heard about cohere re ranking service, or this kind of ranking services where you basically input the query and all the documents, and they go and score everything. But then you have everything in memory already. Retrieve the documents, and imagine doing that at the web scale, or if you have 100 million documents is is not possible, right? Jo: And it's also similar to doing a graph. So instead, we would like to have some kind of technique for representing these documents so that we can index them, so that when the query comes in that we efficiently can retrieve over this representation, and that we efficiently in sublinear time can retrieve the kind of top rank docs, and then we can feed that into subsequent ranking faces. Jo:這種表示方法的動機是你想避免對集合中的所有文檔進行評分。
Jo:所以如果你在使用,有些人可能聽說過 cohere 重新排序服務,或者這種排序服務,你基本上輸入查詢和所有文檔,然後它們會對所有東西進行評分。但這樣你已經把所有東西都放在記憶體中了。檢索文檔,想像一下在網頁規模上這樣做,或者如果你有一億個文檔,這是不可能的,對吧?
Jo:這也類似於做圖表。所以,我們希望有某種技術來表示這些文檔,以便我們可以對它們進行索引,這樣當查詢進來時,我們可以有效地檢索這些表示,並且我們可以在次線性時間內有效地檢索到排名靠前的文檔,然後我們可以將其輸入到後續的排序階段。 | 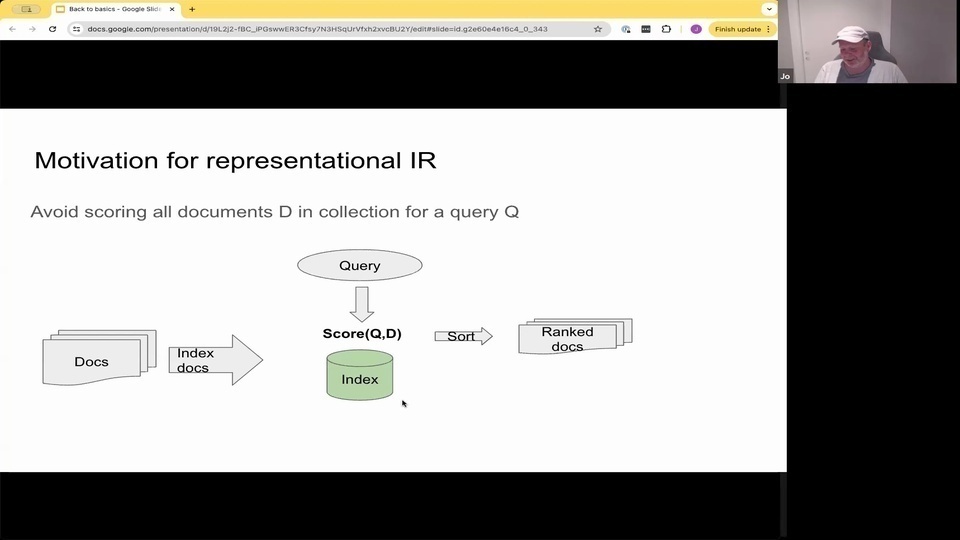 圖片編號: 329 圖片時間: 1640.00秒 |
| 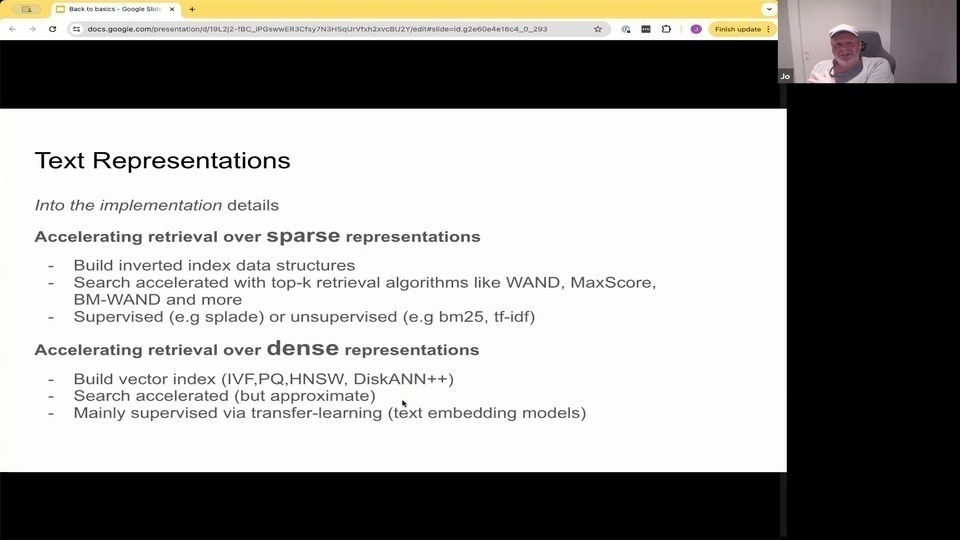 圖片編號: 332 圖片時間: 1655.00秒 |
Jo: And there are 2 primary representations. And that is the sparse representation where we basically have the total vocabulary is kind of the whole sparse vector representation that you potentially take what for a given query or a given document? Jo: Only the words that are actually occurring in that document or in that query, have a non 0 weight. Jo: and this can be efficiently retrieved over using algorithms like weekend or Max score and inverted indexes. You're all familiar with elastic search or other kind of keyword search technologies. They build on this. More recently, we also have using neural or kind of embedding or sparse embedding models, so that instead of having a unsupervised way that is just based on your corpus statistics. You can also use transformer models to Jo: learn the weights of the words in the queries on the documents. Jo: and then you have dense representations. And this is where you have text embedding models, where you take some text and you encode it into this latent embedding space. And you compare queries and documents in this latent space, using some kind of distance metric. Jo: And there you can build indexes using different techniques, vector databases, different types of algorithms. And in this case also, you can accelerate search quite significantly so that you can search even 1 billion scale data sets in milliseconds single credit Jo: but the downside is that there are a lot of trade offs related to that the actual search is not exact. It's an approximate search, so you might not retrieve exactly the ones that you would do if you did. A brute force search over all the vectors in the collection. Jo: And these representations are mainly Jo: supervised through transfer learning. Because you using typically an off the shelf embedding models that's been trained on some other data, some data sets. And then you're trying to apply that to your model. You can fine tune it if you have relevancy data and so forth. Then it's no longer like a 0 shot or transfer learning, but still like a learn representation. Jo: And I think these representations and the whole chat gpt open AI chat Gpt language model open AI Embeddings really open the world of embeddings to a lot of developers. And this stock for quite some time. And it's still stuck, I think, because people think that this will give you a magical AI powered representation. Jo:有兩種主要的表示方法。第一種是稀疏表示法,基本上我們有一個完整的詞彙表,這個詞彙表是稀疏向量表示法,你可以用它來處理給定的查詢或文檔。 Jo:只有實際出現在該文檔或查詢中的詞語才會有非零權重。 Jo:這可以通過使用像 weekend 或 Max score 和倒排索引這樣的算法來高效檢索。你們都熟悉 elastic search 或其他類型的關鍵詞搜索技術,它們都是基於這個原理。最近,我們也開始使用神經網絡或嵌入模型,稀疏嵌入模型,這樣就不再是僅僅基於語料庫統計的無監督方法。你還可以使用 transformer 模型來 Jo:學習查詢和文檔中詞語的權重。 Jo:然後你有密集表示法。這是你使用文本嵌入模型的地方,你將一些文本編碼到這個潛在的嵌入空間中。然後你使用某種距離度量在這個潛在空間中比較查詢和文檔。 Jo:在這裡,你可以使用不同的技術來構建索引,向量數據庫,不同類型的算法。在這種情況下,你也可以顯著加速搜索,甚至可以在毫秒級別內搜索十億級別的數據集。 Jo:但缺點是有很多相關的權衡,實際搜索並不精確。這是一種近似搜索,所以你可能無法檢索到你在對整個集合中的所有向量進行暴力搜索時會檢索到的精確結果。 Jo:這些表示主要是 Jo:通過遷移學習來監督的。因為你通常使用的是一個現成的嵌入模型,這個模型是在其他數據集上訓練的。然後你試圖將其應用到你的模型中。如果你有相關性數據,你可以對其進行微調等等。這樣它就不再是零樣本或遷移學習,而是一種學習表示。 Jo:我認為這些表示和整個 chat gpt open AI chat Gpt 語言模型 open AI 嵌入模型確實向許多開發者開放了嵌入的世界。這個話題已經討論了相當長的時間。我認為它仍然存在,因為人們認為這會給你一個神奇的 AI 驅動的表示。 | 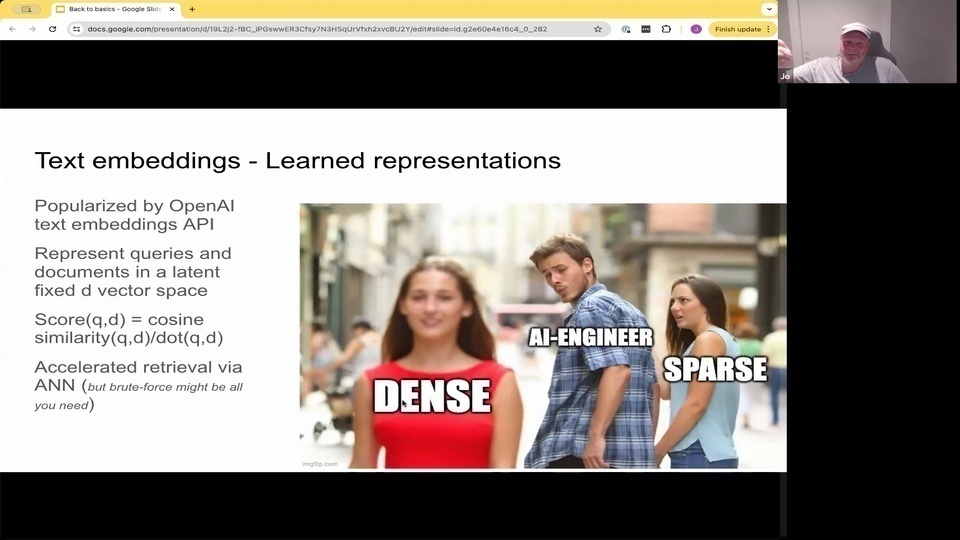 圖片編號: 360 圖片時間: 1795.00秒 |
Jo: It's not bad. And you could also use now a lot of different technologies for implementing search vector databases, regular databases. Everybody now has a vector search report. Which is great because you can now use different or more Jo: wide landscape of of different technologies to kind of solve search. Jo: And but there are some challenges with these text embedding models, especially because the way they work. Jo:『這不錯。而且你現在也可以使用很多不同的技術來實現搜索向量數據庫、常規數據庫。現在每個人都有一個向量搜索報告。這很棒,因為你現在可以使用不同或更多的技術來解決搜索問題。』
Jo:『但是這些文本嵌入模型存在一些挑戰,特別是因為它們的工作方式。』 | 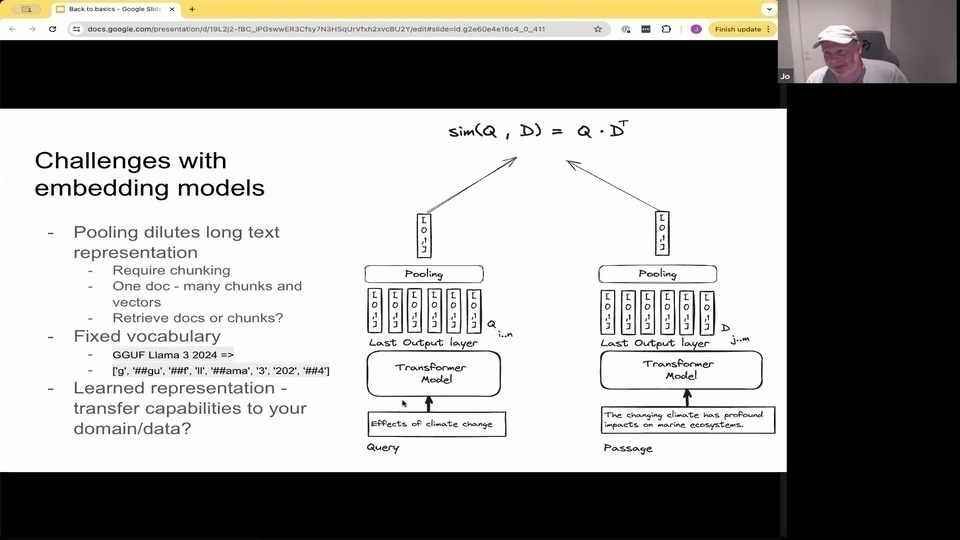 圖片編號: 369 圖片時間: 1840.00秒 |
Jo: Most of them are based on a kind of encoder style transformer model. Where you take the input text, you tokenize it into a fixed vocabulary. Jo: and then you have previously, in the pre-training stage and the fine tuning stage. You have a learn representations of each of these fixed tokens. Jo: Then you feed them through the encoder network. Jo: And for each of the input tokens. Jo: you have an output. Vector Jo: and then there's a pooling step. Jo: typically averaging Jo: into a single vector representation. So this is how you represent not only one word, but a full sentence, or even now, with the embedding model coming out today, supporting, you know, encoding several books as one vector Jo: but Jo: the issue with this is that the representation becomes quite diluted. When you kind of average everything into one vector which is proven not to work that well for high precision search. Jo: So you have to have some kind of shunking mechanism to in order to have a better representation for search. And this fixed vocabulary, especially for birth, based models. You're basing it off a vocabulary that was trained in 2,018. So there are a lot of words that it doesn't know. So we had one issue here with a user that was searching for our recently announced support for running inference with Ggf. Models in Vespa. Jo: And this has a lot of out of word. Oh, sorry out of vocabulary words. So it gets maps to different concepts, and this might produce quite weird results when you are mapping this into the latent embedding space. Jo: And then is the final question is, is, does this actually transfer to your data to your queries? So? And but the framework or the kind of evolution routines that I talked about earlier. Jo: We'll give you the answer to that, because then you can actually test. You know, if if they're working or not. Jo: And Jo: and also, I think, on on the baselines. It's quite important to establish kind of some baselines. And in the information retrieval community, the kind of de facto baseline is. Bm, 25. Jo: So, bm, 25 is this scoring function where you tokenize the text, linguistic processing and so forth. It's well known, implemented in multiple mature technologies like elastic Vespa tantiv whatnot. I think there was a even a library announced today. Bm, 25 in Python. Jo:大部分都是基於一種編碼器風格的 transformer 模型。你將輸入文本分詞成固定詞彙表中的詞彙。
Jo:然後在預訓練階段和微調階段,你已經學習了這些固定詞彙的表示。
Jo:接著你將它們輸入編碼器網絡。
Jo:對於每個輸入的詞彙。
Jo:你會得到一個輸出向量。
Jo:然後有一個池化步驟。
Jo:通常是平均化。
Jo:成為一個單一的向量表示。所以這就是你如何表示不僅是一個詞,而是一整個句子,甚至現在隨著嵌入模型的出現,支持將幾本書編碼成一個向量。
Jo:但是。
Jo:這樣做的問題是,當你將所有東西平均化成一個向量時,表示會變得相當稀釋,這已被證明對於高精度搜索效果不佳。
Jo:所以你必須有某種分塊機制,以便為搜索提供更好的表示。而這個固定詞彙表,特別是對於基於 BERT 的模型,你是基於 2018 年訓練的詞彙表。所以有很多詞它不知道。我們這裡有一個用戶遇到的問題,他在搜索我們最近宣布的在 Vespa 中運行推理的 Ggf 模型支持。
Jo:這裡有很多超出詞彙表的詞。所以它會映射到不同的概念,這可能會在將其映射到潛在嵌入空間時產生相當奇怪的結果。
Jo:最後的問題是,這是否實際轉移到你的數據和查詢中?所以?但是我之前提到的框架或演化路徑。
Jo:會給你答案,因為你可以實際測試它們是否有效。
Jo:而且,我認為在基準線上,建立一些基準線是相當重要的。在信息檢索社區中,事實上的基準線是 BM25。
Jo:所以,BM25 是一個打分函數,你將文本分詞,進行語言處理等等。它是眾所周知的,實現在多個成熟技術中,如 Elastic、Vespa、Tantiv 等等。我認為今天甚至有一個庫宣布了在 Python 中的 BM25。 | 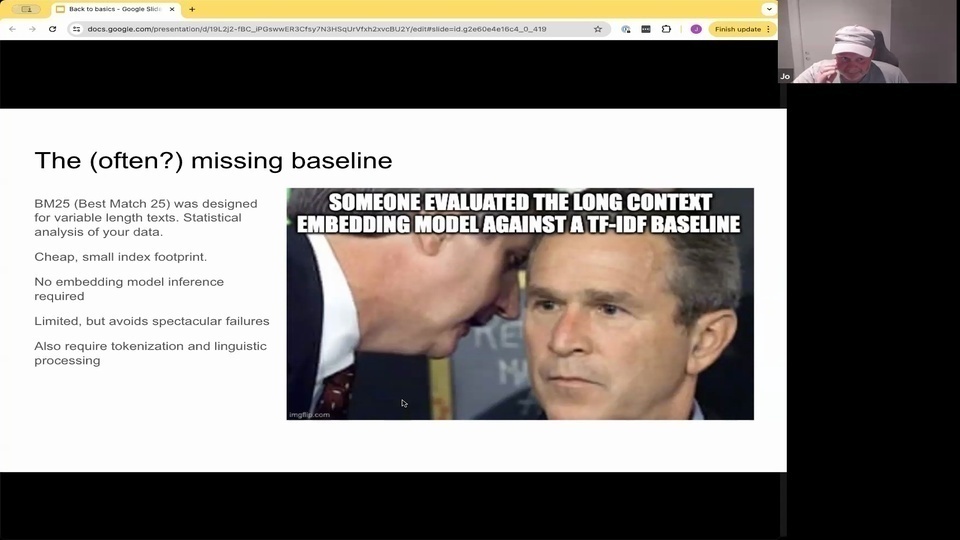 圖片編號: 403 圖片時間: 2010.00秒 |
Jo: So, and and it builds a model kind of model, unsupervised from your data, looking at the words that are occurring in the collection, how many times it's occurring in the data, and and how frequent the word is in in the total collection. And this is scoring function. Jo: and it's very cheap, small index footprint. And most importantly, you don't have to invoke kind of a transformer embedding model like a 7 b llama model, or something like that which is quite expensive. Jo: It has limitations, but it can avoid these kind of spectacular failure. Cases of of embedding retrieval related to out of vocabulary words. Jo: The huge downside is that if you want to make this work in Cjk languages, or or Turkish or different type of languages. You need to have some kind of tokenization integrated which you will find in in engines like elastic search or open search, or or vespa Jo:所以,它會從你的數據中建立一種無監督的模型,查看集合中出現的詞語,這些詞語在數據中出現的次數,以及這些詞語在整個集合中的頻率。這就是評分函數。 Jo:而且它非常便宜,索引佔用空間小。最重要的是,你不需要調用像 7 b llama 模型這樣的 transformer 嵌入模型,這樣的模型相當昂貴。 Jo:它有局限性,但它可以避免嵌入檢索中與詞彙表外詞語相關的這些驚人的失敗案例。 Jo:最大的缺點是,如果你想在 Cjk 語言、土耳其語或其他類型的語言中使用這個方法,你需要集成某種分詞技術,這些技術可以在 elastic search、open search 或 vespa 等引擎中找到。 | 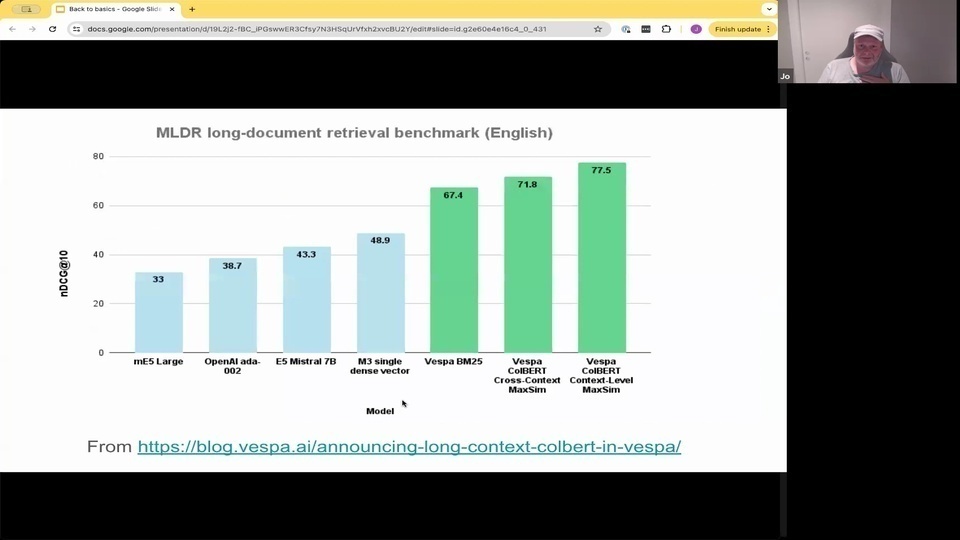 圖片編號: 415 圖片時間: 2070.00秒 |
Jo: and long context. So we did the announcement earlier this year of supporting Colbert in a specific way. I'm just including this to Jo: show you that this is a long context. Documents so they are. I think they're around 3 k tokens long, and the researchers evaluated these different models, and they were presenting results about M. 3, which is scoring 48.9 in this diagram, and they were comparing it with Openai embeddings with M different types of Mistral or different types of embedding models. Jo: And then we realize that you know, this is actually quite easy to beat. Jo: just using a vanilla. Bm, 25. Implementation, even Lucine or vespa, or elasticsearch, or open search. So. Jo: having that kind of mindset that you can evaluate and actually see what works. And remember that beyond 25 can be a strong baseline. I think that's an important takeaway. Jo: Then there's a hybrid alternative. We saw, you see, a lot of enthusiasm around that where you can combine these representations, and it can overcome this kind of fixed vocabulary issue with regular embedding models. Jo:和長上下文。所以我們在今年早些時候宣布以特定方式支持 Colbert。我只是包括這個來 Jo:向你展示這是一個長上下文。文件,所以它們是。我認為它們大約有 3k 個 tokens 長,研究人員評估了這些不同的模型,他們在展示 M.3 的結果,這在這個圖表中得分為 48.9,他們將其與 Openai 嵌入和不同類型的 Mistral 或不同類型的嵌入模型進行比較。 Jo:然後我們意識到,你知道,這其實很容易超越。 Jo:只需使用一個普通的 Bm25 實現,甚至是 Lucine 或 vespa,或 elasticsearch,或 open search。所以。 Jo:擁有那種可以評估並實際看到什麼有效的心態。並記住,bm25 可以是一個強大的基線。我認為這是一個重要的收穫。 Jo:然後有一個混合替代方案。我們看到,您看到,對此有很多熱情,您可以結合這些表示,它可以克服常規嵌入模型的這種固定詞彙問題。 | 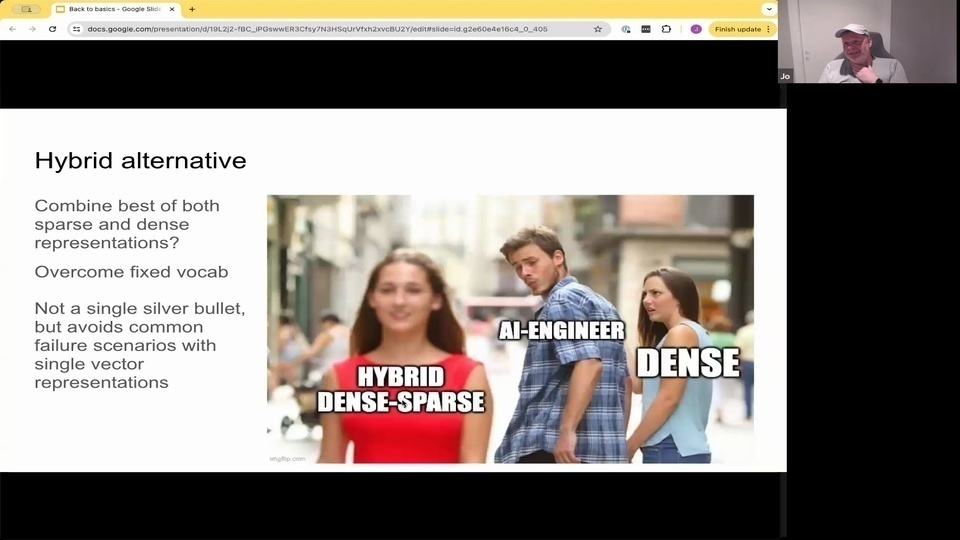 圖片編號: 428 圖片時間: 2135.00秒 |
Jo: But it's not also us. Not a single silver bullet reciprocal rank fusions or methods to fuse this kind of different methods, you know, it really depends on the data and the type of queries. But if you have, build your own emails. Jo: then you don't have to listen to me about. You know what you should do, because you can actually evaluate and test things out, and you can iterate on it. So I think that's that's really critical to be able to build better rag, to be to to improve the quality of the retrieval phase. Jo:但這也不只是我們。沒有一個萬能的解決方案或方法來融合這種不同的方法,你知道,這真的取決於數據和查詢的類型。但如果你有,建立你自己的電子郵件。 Jo:那麼你就不必聽我說你應該做什麼,因為你可以實際評估和測試,並且可以反覆改進。所以我認為這對於能夠建立更好的 RAG,提升檢索階段的質量是非常關鍵的。 | 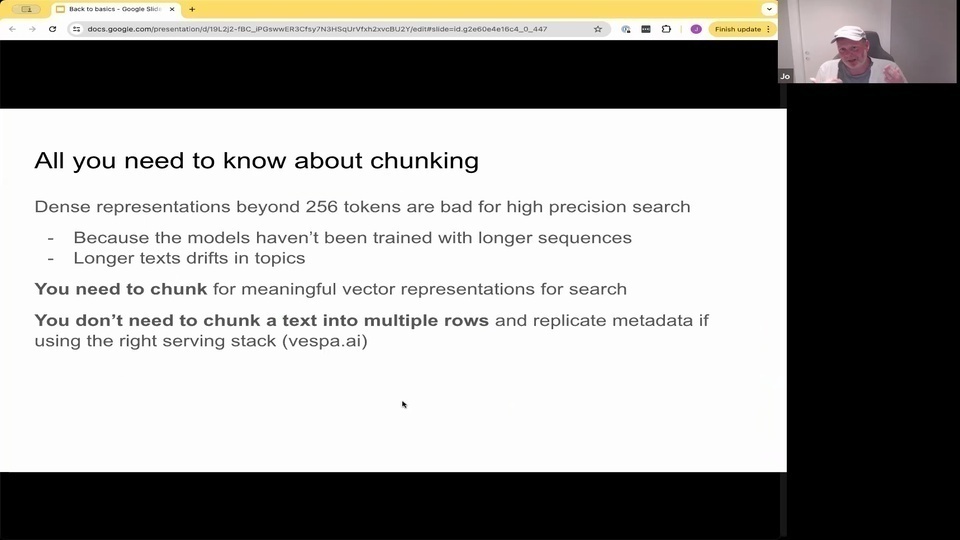 圖片編號: 438 圖片時間: 2185.00秒 |
Jo: Yeah. And of course, I talked about long context. Jo: and that the long context models we all want to get rid of chunking. We all want to get rid of all the videos about how to chunk but Jo: the basic kind of short answer to this is that you do need to chunk in order to have meaningful representations of text for high precision search. Jo: So typically, like Nils rhymers, the de facto embedding expert says that if you go about 250, so 256 tokens. You're starting to lose a lot of precision right? There's other use cases that you can use this embeddings for, like classification. You know, there are a lot of different things. But for high position search it becomes very diluted because of these pooling operations. And also there's not that many great data sets that you can actually train models on have longer text. Jo: And even if you're chunking to have meaningful representation, it doesn't mean that you have to split this into multiple rows in your database. There are technologies that allows you to kind of index multiple vectors per row so that's possible. Finally, real world rag. Not sure. But if you've seen this. But there was a huge Google leak earlier Jo: in in May. Jo: where they revealed a lot of different signals. And in the real world Jo: it's in the real world search. It's more. It's more about not only about the text similarity. It's not only about bm, 25 or a single vector cosine similarity. There are things like freshness, authority, quality patrons you heard about and also revenue. So there are a lot of different features. Jo:對,當然,我談到了長上下文。 Jo:而且我們都希望長上下文模型能夠擺脫分塊。我們都希望擺脫所有關於如何分塊的視頻,但 Jo:簡單的答案是,你確實需要分塊才能對文本進行高精度檢索的有意義表示。 Jo:所以通常來說,就像嵌入專家 Nils Rhymers 說的那樣,如果你超過 250,256 個 tokens,你就會開始失去很多精度,對吧?還有其他用例可以使用這些嵌入,比如分類。你知道,有很多不同的事情。但對於高精度檢索來說,由於這些池化操作,它變得非常稀釋。而且也沒有那麼多優秀的數據集可以用來訓練具有更長文本的模型。 Jo:即使你在分塊以獲得有意義的表示,也不意味著你必須將其拆分成數據庫中的多行。有一些技術允許你在每行中索引多個向量,所以這是可能的。最後,現實世界中的 RAG。不確定你是否見過這個。但在五月初有一個巨大的 Google 洩漏事件 Jo:他們透露了很多不同的信號。在現實世界中 Jo:在現實世界的搜索中,這不僅僅是關於文本相似性。不僅僅是關於 BM25 或單一向量的余弦相似性。還有新鮮度、權威性、質量模式你聽說過的,還有收入。所以有很多不同的特徵。 | 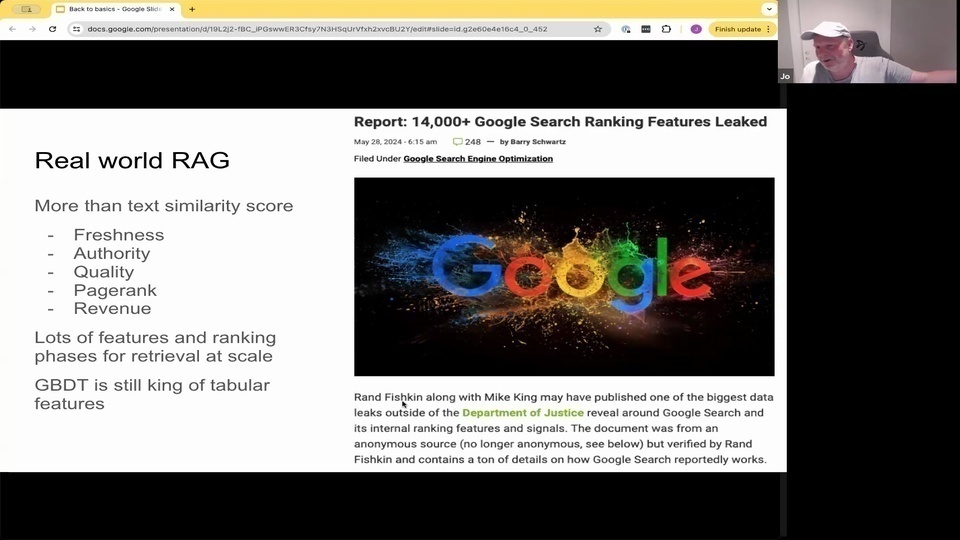 圖片編號: 458 圖片時間: 2285.00秒 |
Jo: and Gbt is still, you know, a simple, straightforward method, and it's still the kind of king of of tabular features where you have Jo: specific name features, and you have values for them. So combining Gbt with this kind of new neural features is quite effective when you're starting to actually operate in the in the real world. Jo: So quick summary. I think that information retrieval is more than just a single vector representation. Jo: And if you want to improve your retrieval stage, you should look at building your own emails. Jo: And please don't ignore the Bm 25 baseline Jo: and choosing some technology that has hybrid capabilities, meaning that you can have Jo:而 Gbt 仍然是一種簡單、直接的方法,它在處理表格特徵時仍然是王者,當你有具體的名稱特徵並且有相應的值時。將 Gbt 與這種新的神經特徵結合起來,在實際操作中是相當有效的。
Jo:所以快速總結一下。我認為信息檢索不僅僅是一個單一的向量表示。
Jo:如果你想改進你的檢索階段,你應該考慮建立你自己的電子郵件系統。
Jo:請不要忽視 Bm 25 基線。
Jo:並選擇一些具有混合能力的技術,這意味著你可以擁有。 | 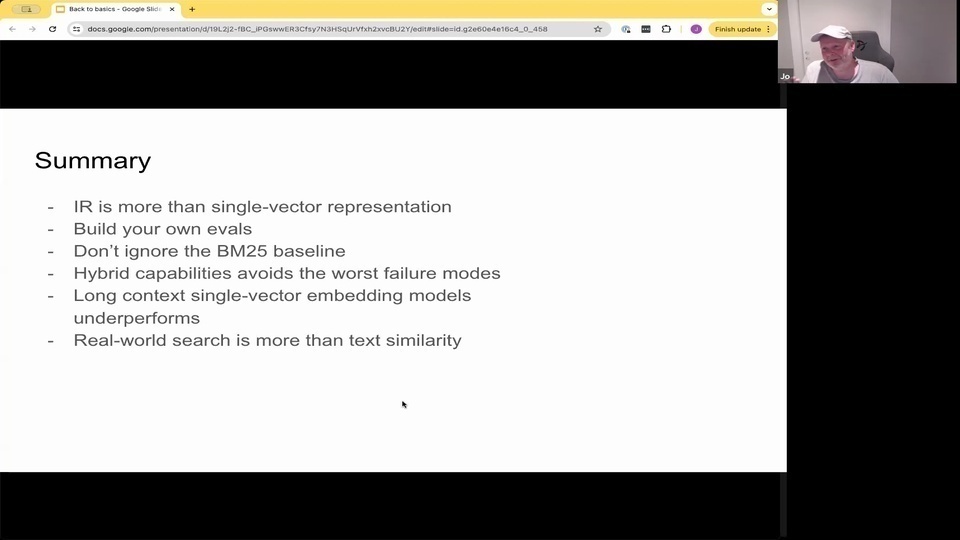 圖片編號: 470 圖片時間: 2345.00秒 |
Jo: exact search for the exact tokens and still have matches, and also combine the signals from Jo: text search via keywords and text search via embeddings Jo: can avoid some of these failure modes that I talked about. Jo: And Jo: yeah. And finally, real-world search is is more than than text similar. Jo:精確搜索精確的標記並仍然有匹配,還可以結合來自 Jo:關鍵詞文本搜索和嵌入文本搜索的信號 Jo:可以避免我提到的一些失敗模式。 Jo:而且 Jo:是的。最後,現實世界的搜索不僅僅是文本相似。 | 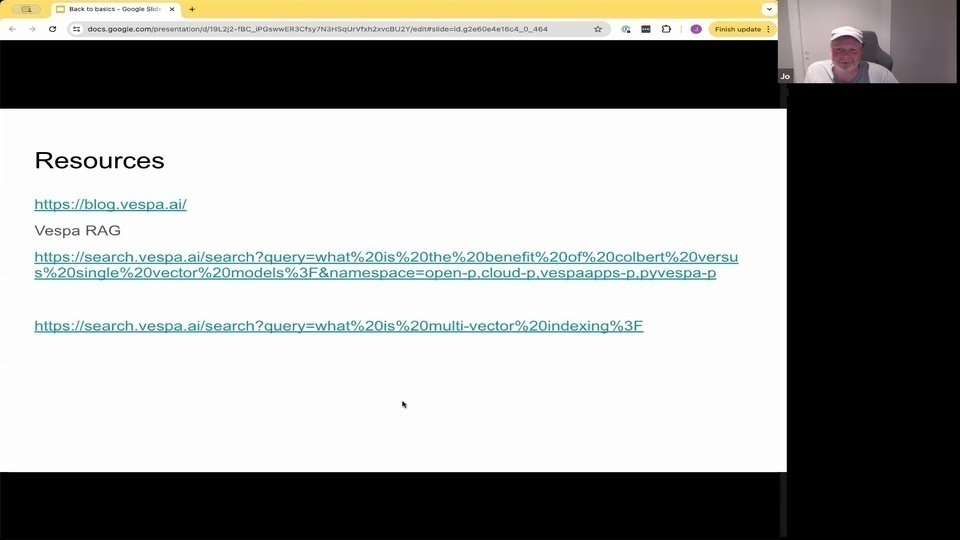 圖片編號: 476 圖片時間: 2375.00秒 |
Jo: So that's what I had. And I hoping for questions. If you wanna check out some resources, I I do a lot of writing on the the blog west by AI, so you can check that out and Jo: if you hated it, you can tweet me at Joe Bergam at Twitter. Jo: I'm quite active there. So I appreciate if you headed it. And since then you can mention me there. And yeah, and you can also contact me on on Twitter. I love getting questions. So. Hamel Husain: That's a that's a bold call to action. If you hated it. Jo: It is. Jo:所以這就是我的分享。我希望能有一些問題。如果你想查看一些資源,我在 west by AI 的部落格上寫了很多文章,所以你可以去看看。 Jo:如果你不喜歡,可以在 Twitter 上推文給我,@Joe Bergam。 Jo:我在那裡非常活躍。所以如果你不喜歡,請告訴我。你也可以在 Twitter 上聯繫我。我很喜歡收到問題。 Hamel Husain:這是一個大膽的行動呼籲。如果你不喜歡。 Jo:是的。 | 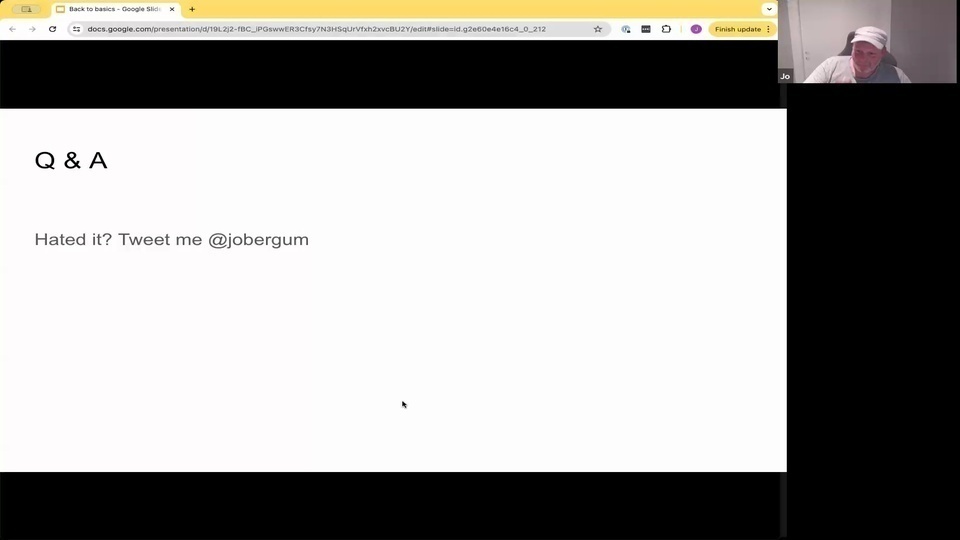 圖片編號: 484 圖片時間: 2415.00秒 |
Hamel Husain: We definitely have a lot of questions. I'll just go through some of them. Jo: Food. Hamel Husain: What kind of metadata is most valuable to put into a vector dB Hamel Husain: for doing rec. Jo: Yeah, if if you look at the only the text domain if you're only concerned about text. So you have no freshness component, or you don't have any authority. If you, for example, are building like a healthcare Jo: or a health, your users are asking helps questions like you definitely wanna have some kind of filtering. What's the authoritative sources within hop health? You don't wanna drag up Reddit or things like that? Right? So, and title and other metadata of courses. But it really depends on the use case if you're like a text, only use case, or if it's like more like real world where you have different types of signals. So. Hamel Husain: Makes sense. Hamel Husain: Do you have any thoughts on calibration of different indices of the different indices? Not only are different document indices not aligned in terms of similarity scores. But it's also nice to have confidence scores, for how likely the recommendation is to be good. Jo: Yeah, I think, is, is a very tough question so these different methods for all these different scoring function you can call that have a different distribution, different shape, different score ranges. So it's really hard to Jo: combine them, and they're not probabilities as well. So it's very difficult to to map them into a probability that actually, this is is, or or filtering people wants to like. Oh, I have a cosine similarity filter on 0 point 8 but it's different from different types of model, but combining them Jo: is also a learning task. It also kind of you need to learn the parameters. And Gbt is quite good at that, because you're learning a nonlinear combination of these different features. But in order to do that, then you also have this have trading data Jo: but the way I described here for doing evaluation can also help you Jo: generate training data for Jo: training ranking models. So. Hamel Husain: So does the calibration really turn into a hyper parameter tuning exercise with your email set? Or does that kind of. Jo: Yeah, well, you you could do that right if if if you don't have, if you don't have any data that you can train them all along to train those parameters. You could do hyper parameter sweep. And then, you know, basically check if your Eval is improving or not. Jo: But if you wanna apply like more like a Ml technique on this right then you will either, like Google is doing like gathering search and clicks and interactions. But now we also see more that people actually using large language models to generate syntactic training data. So you can distill kind of the the powers of the the larger models into smaller models that you can use for for ranking purposes, but is is a very broad topic. I think Jo: so. It's very difficult to kind of deep dive. And it is very difficult to say that. Oh, you should have a cut off 0 point 8 on the vector similarity. And or you can do this transformation. So there, there are no like really great tricks to to do this. Without having some kind of training data, and at least some evaluations. Hamel Husain: What are your observations on Hamel Husain: the efficacy of rerankers? And do you usually recommend to use a reranker. Jo: Yeah, because the rerankers the the the great thing about rerankers is that in the face between people and ranking pipelines, you're gradually throwing away hits. Using this kind of representational approach, and then you can have a gradual approach where you're investing more compute into fewer hits and still be within your latency budget. And then the great thing about rerankers like coherent. You could deploy them. Investment as well Jo: is that they offer this kind of token level interaction. Jo: Because you input both the query and the document at the same time through the transformer network. And then you have token level interactions. You're no longer interacting between the query and the document through this vector representation. But you're actually interi feeding all the tokens of the query and the document into the method. So yeah, that definitely can can help accuracy. But that is coming a question about cost and latency and so forth. Jo: Yeah, so a lot of tradeoffs in in this. But if you're only looking at accuracy and you can afford the additional cost, yeah, definitely, they they can help. Hamel Husain:我們確實有很多問題。我會先回答其中一些問題。
Jo:食物。
Hamel Husain:什麼樣的元數據最有價值放入向量資料庫來做推薦?
Jo:是的,如果你只關注文本領域,你只關心文本。所以你沒有新鮮度組件,或者你沒有任何權威性。如果你,例如,正在構建一個醫療保健或健康系統,你的用戶在問健康問題,你肯定想要有某種過濾。什麼是健康領域內的權威來源?你不想拖出 Reddit 或類似的東西,對吧?所以,標題和其他元數據當然也很重要。但這真的取決於使用案例,如果你是純文本使用案例,或者是更像現實世界的使用案例,你有不同類型的信號。
Hamel Husain:有道理。
Hamel Husain:你對不同索引的校準有什麼看法?不同的文檔索引在相似度分數方面不一致,但擁有信心分數也是很好的,這樣可以知道推薦的可能性有多大。
Jo:是的,我認為這是一個非常棘手的問題,因為這些不同的評分方法有不同的分佈、不同的形狀、不同的分數範圍。所以很難將它們結合起來,而且它們也不是概率。因此,很難將它們映射到一個實際的概率上,或者過濾人們想要的東西。哦,我有一個 0.8 的餘弦相似度過濾器,但這對不同類型的模型來說是不同的,但將它們結合起來也是一個學習任務。你需要學習參數。而 Gbt 在這方面相當不錯,因為你在學習這些不同特徵的非線性組合。但為了做到這一點,你還需要有訓練數據。
Jo:但我在這裡描述的評估方法也可以幫助你生成訓練數據來訓練排序模型。
Hamel Husain:那麼校準真的變成了一個超參數調整的練習嗎?
Jo:是的,你可以這麼做,如果你沒有任何數據可以用來訓練這些參數,你可以進行超參數掃描。然後,基本上檢查你的評估是否在改進。
Jo:但如果你想應用更多的機器學習技術,像 Google 那樣收集搜索和點擊以及交互數據。但現在我們也看到更多的人實際上使用大型語言模型來生成合成訓練數據。這樣你可以將大型模型的能力提取到較小的模型中,用於排序目的,但這是一個非常廣泛的話題。我認為很難深入探討。很難說你應該在向量相似度上設置 0.8 的截止點,或者你可以進行這種轉換。所以,沒有真正的好方法來做到這一點,除非你有一些訓練數據,至少有一些評估。
Hamel Husain:你對重新排序器的有效性有什麼觀察?你通常建議使用重新排序器嗎?
Jo:是的,因為重新排序器的好處是,在人們和排序管道之間,你逐漸丟棄命中結果。使用這種表示方法,你可以逐步投入更多的計算資源到更少的命中結果中,並且仍然在你的延遲預算內。重新排序器的好處是,你可以部署它們,這也提供了這種令牌級別的交互。
Jo:因為你同時將查詢和文檔輸入到 transformer 網絡中,然後你有令牌級別的交互。你不再通過向量表示在查詢和文檔之間進行交互,而是實際上將查詢和文檔的所有令牌輸入到方法中。所以,是的,這肯定可以幫助提高準確性。但這涉及到成本和延遲等問題。
Jo:是的,這裡有很多權衡。但如果你只關注準確性並且能夠承擔額外的成本,是的,它們肯定可以幫助。 | 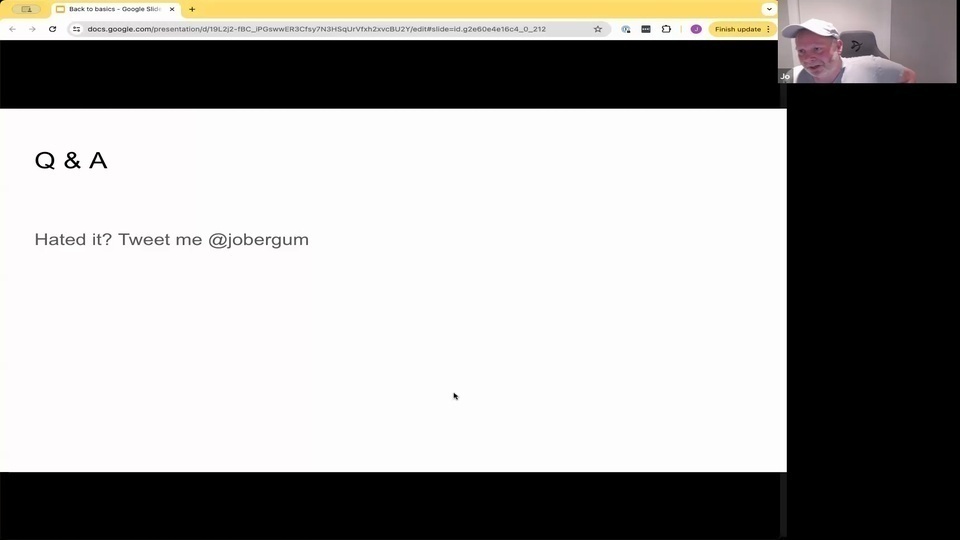 圖片編號: 538 圖片時間: 2685.00秒 |
Jo: Yep. Hamel Husain: Hey, William Horton is asking, do you have advice on combining usage data along with semantic similarity, like, if I have a number, then, like a number of views or some some kind of metadata like that from a document. Hamel Husain: Yeah, I want to say. Jo: Goal is. Hamel Husain: To, the. Jo: It goes. Yeah, it goes into more of if you have interaction data, it becomes more of a learning to rank problem. Jo: You 1st need to come out with labels from those interaction because there'd be gonna be multiple interactions. And then you, there's gonna be add to charge at different different actions will have different weights. So the standard procedure is that you, you convert that the data into kind of a label data set similar to what I shown here in the Eval Jo:對。
Hamel Husain:嘿,William Horton 問,你有沒有關於結合使用數據和語義相似性的建議,比如,如果我有一個數字,然後,比如來自文檔的一些瀏覽次數或某種元數據。
Hamel Husain:是的,我想說。
Jo:目標是。
Hamel Husain:要,這個。
Jo:它是這樣的。是的,如果你有互動數據,它就變成了一個學習排序問題。
Jo:你首先需要從那些互動中得出標籤,因為會有多次互動。然後,你會有不同的動作會有不同的權重。所以標準程序是,你將數據轉換成類似於我在這裡展示的 Eval 中的標籤數據集。 | 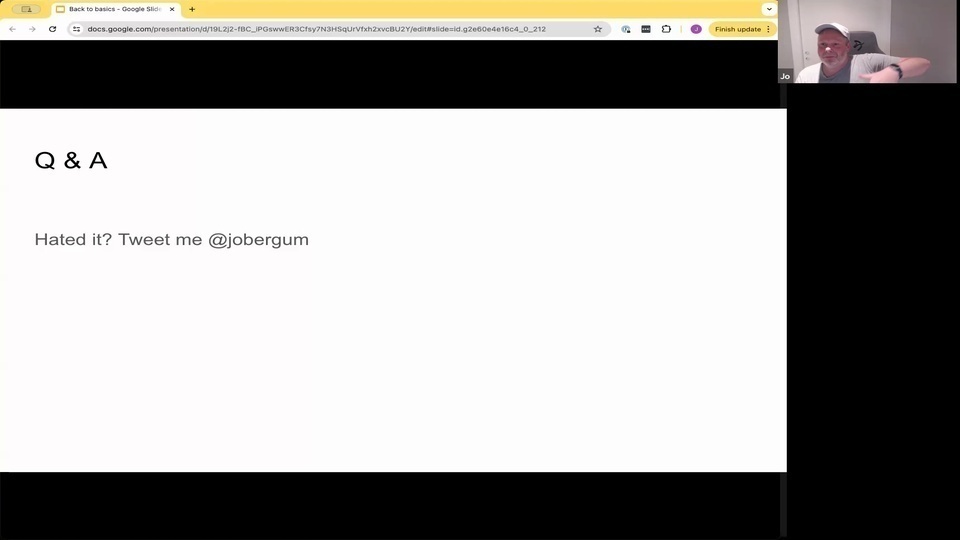 圖片編號: 549 圖片時間: 2740.00秒 |
Jo: so when you convert that to kind of a label data set, then you can train a model, for instance, a Gbt model where you can include Jo: the semantic score as well as a feature. Jo: Yeah. Hamel Husain: Alright. Someone's asking the question Hamel Husain: that you may not be familiar with. But I was, gonna give it a shot. It's a reference to someone else. What are your thoughts on Jason Lou's post about the value of generating structured summaries and Hamel Husain: reports for decision makers, instead of doing rag the way we are doing. Leslie's commonly done today. Have you seen seen that you, familiar with. Jo: Yeah, I mean, Jason is fantastic. I I love Jason and but he's a high volume Twitter. So I I don't read everything. So i i i haven't. I haven't caught up on that yet. No, sorry. Hamel Husain: Okay, no worries. I don't wanna try to rehash it from my memory either. Jo: Sounds. Hamel Husain: Just skip that one. Hamel Husain: What are some of your favorite advancements recently in text embedding models or other search technologies Hamel Husain: that people Hamel Husain: I'll just stop the question there, yeah, what are your? Hamel Husain: Yep. What? Yeah. Yeah. Jo: Yeah, I I think I think I'm betting Miles will will Jo: be become better. What I do hope is that we can have models that are have a larger vocabulary. Jo: So like llama vocabulary, so we have a larger vocabulary. So like bird models, they have this old vocabulary from 2,018. I think we I would love to see a new kind of Diberta model trained on more recent data, with more recent techniques, like a pre-training stage, including a larger vocabulary for the organization. Jo: I think, as I said, that I'm not Jo: too hyped about increasing the complex length because all the information. Retrieval research shows that Jo: they are not that well, at generalizing a long text into a good representation for high position. Search Jo: so I'm not so excited about the direction where you're going, with just larger, larger, larger, complex windows for embedding models, because I I think it's the wrong direction. I would rather see Jo: larger vocabularies and and better pre trained models like Diberta. It's it's still a good model for for embeddings. Yeah. Hamel Husain: Someone's asking does query expansion of out of vocabulary words with bm, 25. Work better at search. And I think, like, just add onto that. Do you think people are Hamel Husain: going as far with classical search techniques as they should Hamel Husain: like? You know, things like query, expansion, and all kinds of other stuff that have been around for a while before. Jo:所以當你將其轉換為一種標籤數據集時,你可以訓練一個模型,例如一個 Gbt 模型,其中你可以包括語義分數作為一個特徵。
Jo:對。
Hamel Husain:好的。有人在問一個問題
Hamel Husain:你可能不太熟悉。但我想試試看。這是對另一個人的引用。你對 Jason Lou 關於為決策者生成結構化摘要和報告的價值的帖子有什麼看法,而不是像我們現在這樣做 rag。Leslie 今天常做的事情。你有看過嗎,你熟悉嗎。
Jo:是的,我的意思是,Jason 很棒。我我喜歡 Jason,但他是個高產的 Twitter 用戶。所以我我不會讀所有的東西。所以我我我還沒有。我還沒有跟上。抱歉。
Hamel Husain:好的,沒關係。我也不想從我的記憶中重述它。
Jo:聽起來。
Hamel Husain:跳過這個問題。
Hamel Husain:你最近在文本嵌入模型或其他搜索技術方面最喜歡的進展是什麼
Hamel Husain:人們
Hamel Husain:我就停在這裡,對,你的?
Hamel Husain:對。什麼?對。對。
Jo:是的,我我認為我認為我押注 Miles 會會
Jo:變得更好。我希望我們能有一個詞彙量更大的模型。
Jo:所以像 llama 詞彙量,所以我們有一個更大的詞彙量。所以像 bird 模型,它們有這個來自 2018 年的舊詞彙量。我認為我們我希望看到一種新的 Diberta 模型,訓練在更近期的數據上,使用更近期的技術,比如預訓練階段,包括一個更大的詞彙量為組織。
Jo:我認為,正如我所說,我不
Jo:太熱衷於增加複雜的長度,因為所有的信息檢索研究表明
Jo:它們在將長文本概括為高精度搜索的良好表示方面並不那麼好
Jo:所以我對你們走的方向不太興奮,只是更大、更大、更大的複雜窗口用於嵌入模型,因為我我認為這是錯誤的方向。我寧願看到
Jo:更大的詞彙量和更好的預訓練模型,比如 Diberta。它它仍然是一個很好的嵌入模型。對。
Hamel Husain:有人在問,使用 bm25 進行超出詞彙範圍的詞的查詢擴展是否在搜索中效果更好。我認為,僅僅補充一下。你認為人們
Hamel Husain:在使用經典搜索技術方面是否做得足夠
Hamel Husain:比如查詢擴展和其他已經存在一段時間的東西。 | 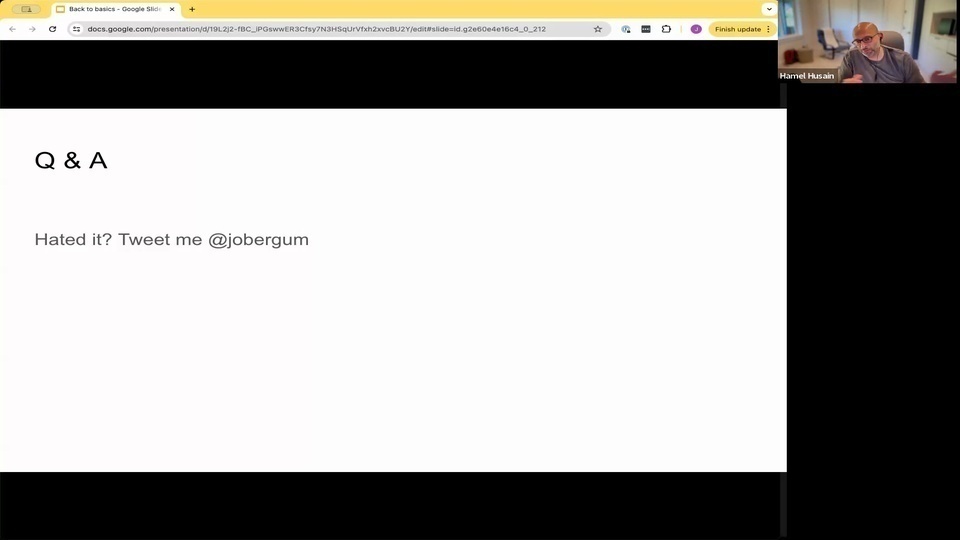 圖片編號: 584 圖片時間: 2915.00秒 |
Hamel Husain: like, what's your feeling about the spectrum? And like, Yeah. Jo: I I think you can get really good results by starting with Bm, 25 and classical resorts, and adding a reranker on top of that. Jo: You won't get the magic if you have a single word query. Jo: and there are no words in your collection. Then you might fail at recall. But you don't get into this kind of really Jo: nasty failure modes of of embedding vector search alone. Jo: And yeah, definitely, there are techniques like query, expansion, query, understanding, and language models. They are also quite good at this. There's a paper from Google. They did query expansions with the Gemini but Jo: pretty well, not amazingly well compared to the size of the model and the additional latency. But we have much better tools for doing query, expansion. All kind of fancy techniques now involving prompting of live language models so Jo: definitely that, too, is is really interesting for expansion. So that's another way. But. Jo: like in the the diagram where. So this machine and all these components and things like that, what I'm hoping people can take away from this is that if you're wondering about this technique that technique I read about this is that Jo: if you put that into practice in a more systematic way, having your own evil. You will be able to answer those questions on your data, on your curs. Without me saying that the the threshold should be 0 point 6 right, which is bullshit, because I don't know your curies or your domain or your data. Jo: So by building these emails, then you can actually iterate and and get the answers. Hamel Husain: In one slide you mentioned limitations and fixed vocabulary with text that is chunked poorly. How do you overcome these sort of limitations in a domain that uses a lot of jargon, and that doesn't tokenize well with an out of the box model. Jo: Yeah, that then you're out of luck with the regular embedding models. And that's why the hybrid capability we actually can combine the keyword search with the embedding retrieval mechanism. But the hard thing is to understand when to completely ignore the embedding results. Jo: because embedding retrieval, no matter how far they are in the out in the vector space will be retrieved right? So when you're asking for 10 nearest neighbors, they might not be so near. But you're still retrieving some junk. Jo: And then it's important to understand that this is actually junk, so that you don't use like techniques like reciprocal rank fusion, which by some vendors, is sold as the the full kind of bone solution to solve all this. But then you're just blending rubbish into something that could be reasonable from the keyword search. So Hamel Husain:像是,你對這個範圍有什麼感覺?然後,嗯。
Jo:我覺得你可以從 Bm25 和經典的 resort 開始,然後在這之上加一個重新排序器,這樣可以得到非常好的結果。
Jo:如果你只有一個單詞查詢,你不會得到魔法。
Jo:而且你的集合中沒有這些詞彙,那麼你可能會在召回上失敗。但你不會陷入嵌入向量搜索單獨使用時那種非常糟糕的失敗模式。
Jo:而且,確實,有一些技術像查詢擴展、查詢理解和語言模型。它們在這方面也相當不錯。Google 有一篇論文,他們用 Gemini 做了查詢擴展,但
Jo:效果還不錯,但相較於模型的大小和額外的延遲,並不是特別驚人。但我們現在有更好的工具來做查詢擴展。所有這些花哨的技術現在都涉及到即時語言模型的提示,所以
Jo:這也是非常有趣的擴展方式。所以這是另一種方法。但是。
Jo:就像在圖表中,這台機器和所有這些組件和類似的東西,我希望人們能從中學到的是,如果你對這種技術感到好奇,我讀過這個技術
Jo:如果你以更系統的方式實踐它,有自己的評估。你將能夠在你的數據、你的查詢上回答這些問題。而不是我說門檻應該是 0.6,這是胡說八道,因為我不知道你的查詢或你的領域或你的數據。
Jo:所以通過構建這些評估,你實際上可以迭代並得到答案。
Hamel Husain:在一張幻燈片中,你提到固定詞彙和文本分塊不佳的限制。你如何在使用大量行話且無法用現成模型進行良好分詞的領域中克服這些限制?
Jo:是的,那麼你在常規嵌入模型中就沒有運氣了。這就是為什麼我們實際上可以將關鍵詞搜索與嵌入檢索機制結合的混合能力。但困難的是要理解何時完全忽略嵌入結果。
Jo:因為嵌入檢索,無論它們在向量空間中有多遠,都會被檢索到,對吧?所以當你要求 10 個最近鄰居時,它們可能並不那麼近。但你仍然會檢索到一些垃圾。
Jo:然後重要的是要理解這實際上是垃圾,所以你不會使用像互惠排名融合這樣的技術,這被一些供應商賣作解決所有這些問題的完整解決方案。但這樣你只是將垃圾混合到可能從關鍵詞搜索中得到的合理結果中。所以 | 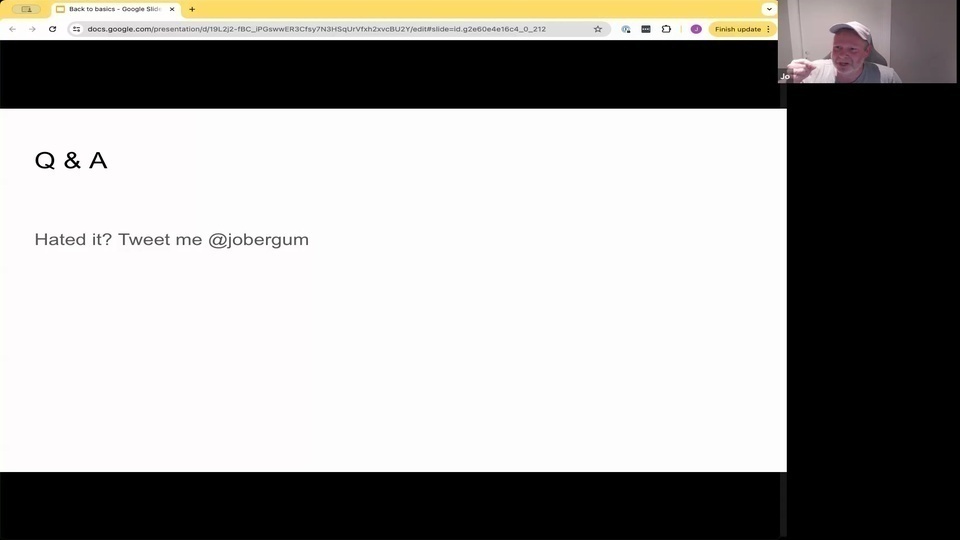 圖片編號: 619 圖片時間: 3090.00秒 |
Jo: currently, and another alternative is as well. That might do a little bit. Stop capping is to fine tune your own embedding model. But you still have the vocabulary issues. So, but if you have resources to kind of, do the pre training stage Jo: on your data with a vocabulary that is more matching up with your domain. That might work. But then you have a training job that goes from scratch. But I hear it's a lot easier to train work from scratch nowadays than in 2,018. So it's it might be a viable option for some organizations. Jo: Most of the companies are doing this. Anyway, they're they're starting in all their semantic search papers. They basically say, here's our pipeline. We pre trained to build a tokenizer on the whole Amazon corpus. Right? They they don't use birth base from 2,018 Jo: right. Hamel Husain: That makes sense. Okay, last question. Hamel Husain: would you see, Colbert, based methods get around or at least improve retrieval when we're concerned with tokenizer problems. Jo: Yeah. So cover to introduce that is basically another neural method where you, instead of learning one vector representation of the full passage or the full query. Jo: you are learning token level vector representations. And this is a bit more expensive. Compute wise at serving time than the regular single embedding models, but Jo: it has close to the accuracy of like the regular rewriteer. Jo: but it's still also suffer from a vocabulary. Because it still uses the same kind of vocabulary as other models. So, but if we can get better Jo: pre-trained model that are trained with a larger vocabulary, I hope that it's a path towards better kind of neural search with Colbert and other embedding models as well. Hamel Husain: Hey? Great? Hamel Husain: Yeah, that's it. There's certainly more questions. But you know we don't want to go on for infinite amount of time. I think we hit the more important ones. Hamel Husain: So yeah, I was a little. Jo: There's there's a lot of great questions. So if you if you wanna, you know. Jo: throw them at me at Twitter, and I'll try to answer as best as possible. Dan Becker: Thanks. Joe. Hamel Husain: Yeah, thank, you. Jo: Yeah. Great being here. Great seeing you guys and have a great day. Jo: Bye, bye.
| |